Review: 1
15 Jul 2022
STAGE 1
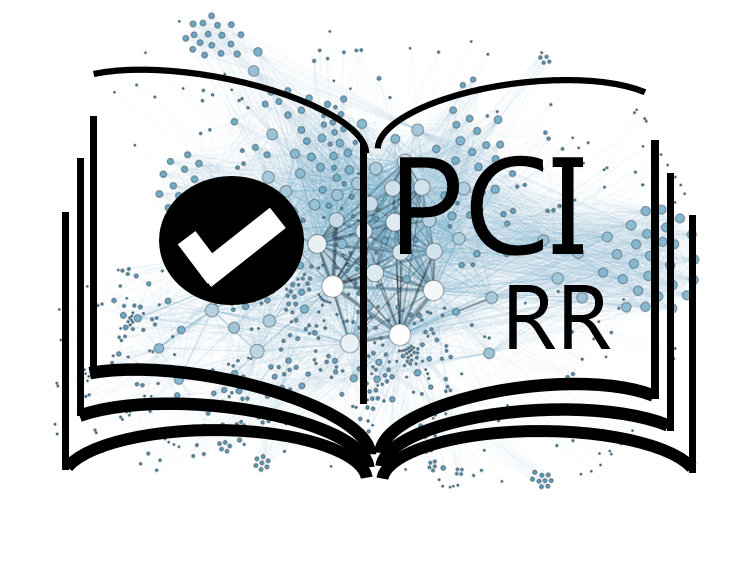
Registered Report: A Laboratory Experiment on Using Different Financial-Incentivization Schemes in Software-Engineering Experimentation
Bug detection in software engineering: which incentives work best?
Recommended by Chris Chambers based on reviews by Edson OliveiraJr and 1 anonymous reviewerBug detection is central to software engineering, but what motivates programmers to perform as optimally as possible? Despite a long history of economic experiments on incentivisation, there is surprisingly little research on how different incentives shape software engineering performance. In the current study, Krüger et al. (2022) propose an experiment to evaluate how the pay-off functions associated with different financial incentives influence the performance of participants in identifying bugs during code review. The authors hypothesise that performance-based incentivisation will result in higher average performance, as defined using the F1-score, and that different incentivisation schemes may also differ in their effectiveness. As well as testing confirmatory predictions, the authors will explore a range of ancillary strands, including how the different incentivisation conditions influence search and evaluation behaviour (using eye-tracking), and the extent to which any effects are moderated by demographic factors.
The Stage 1 manuscript was evaluated over one round of in-depth review. Based on detailed responses to the recommender and reviewers' comments, the recommender judged that the manuscript met the Stage 1 criteria and therefore awarded in-principle acceptance (IPA).
URL to the preregistered Stage 1 protocol: https://osf.io/s36c2
URL to the preregistered Stage 1 protocol: https://osf.io/s36c2
Level of bias control achieved: Level 6. No part of the data or evidence that will be used to answer the research question yet exists and no part will be generated until after IPA.
List of eligible PCI RR-friendly journals:
List of eligible PCI RR-friendly journals:
References
1. Krüger, J., Çalıklı, G., Bershadskyy, D., Heyer, R., Zabel, S. & Siegmar, O. (2022). Registered Report: A Laboratory Experiment on Using Different Financial-Incentivization Schemes in Software-Engineering Experimentation, in principle acceptance of Version 3 by Peer Community in Registered Reports. https://osf.io/s36c2