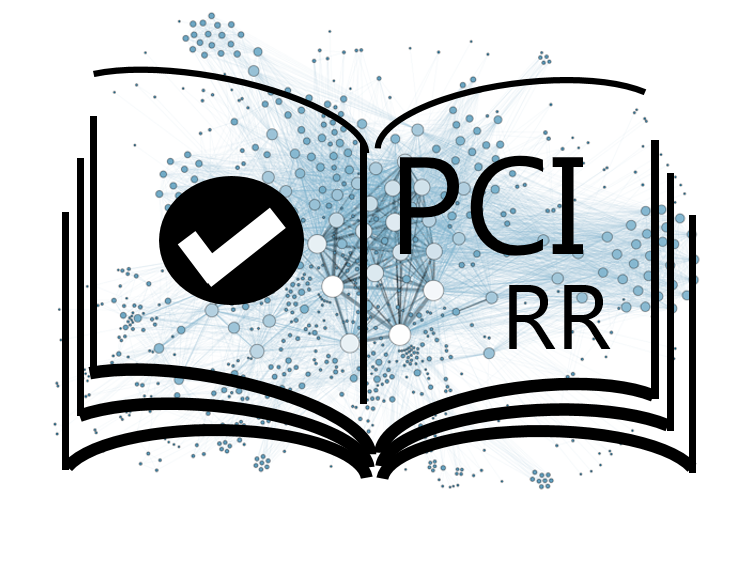
A reliable measure of physical closeness in interpersonal relationships?
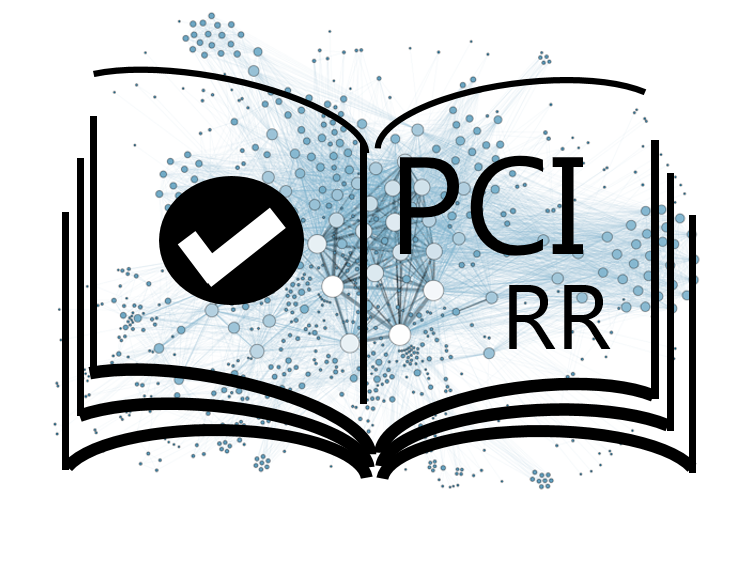
Test-Retest Reliability of the STRAQ-1: A Registered Report
Abstract
Recommendation: posted 17 July 2023, validated 18 July 2023
Syed, M. (2023) A reliable measure of physical closeness in interpersonal relationships? . Peer Community in Registered Reports, . https://rr.peercommunityin.org/PCIRegisteredReports/articles/rec?id=419
Related stage 2 preprints:
Olivier Dujols, Siegwart Lindenberg, Caspar J. van Lissa, Hans IJzerman
https://doi.org/10.31234/osf.io/392g6
Recommendation
Level of bias control achieved: Level 3. At least some data/evidence that will be used to the answer the research question has been previously accessed by the authors (e.g. downloaded or otherwise received), but the authors certify that they have not yet observed ANY part of the data/evidence
List of eligible PCI RR-friendly journals:
The recommender in charge of the evaluation of the article and the reviewers declared that they have no conflict of interest (as defined in the code of conduct of PCI) with the authors or with the content of the article.
Evaluation round #1
DOI or URL of the report: https://psyarxiv.com/392g6
Version of the report: https://psyarxiv.com/392g6
Author's Reply, 30 Jun 2023
Revision & Response letter PCI-RR STRAQ-1 Stage 1
Dear Prof. Moin Syed,
We would like to thank you and the two reviewers for your comments on the manuscript, which directly contributed to improving it. We have revised the manuscript and the analytic code and provided a point-by-point response to the reviewer’s comments. To facilitate the review, we provide a version of the article with Track Changes enabled, and a clean copy without tracking.
Overall, our two biggest changes consisted of (1) better justifying the analytic choices we made in the main text: a) providing greater detail about the procedure, b) providing detailed analytic choices throughout, c) providing specific cut-offs along with their interpretation, and (2) providing reproducible analysis code that now better constrains the planned analysis. In the following, we separate the comments by theme and then by reviewer to make the comments easier to process.
Major Points
Justifying our subjective decisions and improving our analytic code
A measurement project such as this involves many subjective decisions that must be made on issues for which there is no clear answer. The reviewers provide some suggestions for how to resolve the issues that they identified, but in many cases there may be other defensible options. Thus, a general message that you should take from the reviewer comments is that you need to provide much more detail and better justify your decisions throughout. (Moin Syed)
One specific and important issue to attend to is that both reviewers had some trouble accessing your analytic code but in different ways. Please be sure that you are providing the correct links for this Stage 1 review process; having full access to your code will facilitate the review process. (Moin Syed)
Please note that while the OSF project containing the code for the analyses etc is linked in the manuscript, it is currently not public. As such, I couldn't review the code. (Ian Hussey)
Under the link https://osf.io/ab73e/, I could not find the code indicated in the manuscript. I found it here: https://osf.io/mr8n3/. The latter script seems incomplete (by the way: why a pdf not Rmd was submitted?). For instance, it is not clear what package(s) will be used in data analysis – lavaan, I presume. (Jacek Buczny)
Response: We apologize for the unclear links and the incomplete code. We now better justify our decision criteria and cut off values in the manuscript throughout. Please excuse us for the issue with the analytic code. We also provide a public OSF link with the complete planned analytic code. To accommodate this, we completely rewrote the R analysis script in RMarkdown. The code should now be clearer and reproducible. This code reflects our choices that we justify in the manuscript.
About our sample and statistical power
Data loss was substantial. Between 2018 and 2021, more than 1000 participant provided their responses, but only 184 will be included in the analyses. Data loss could be systematic, the sample of 184 may somehow deviate from the subpopulation (students), and thus, the generalizability of the results may be very limited. (Jacek Buczny)
Response: We agree that generalizability is limited, and part of that lack of generalizability could indeed be due to systematic data loss. But that is, of course, not the only constraint on generalizability: our sample consists of psychology students, who mostly identify as women. In the discussion section of the manuscript, and as is our custom, we will commit to adding a “Constraints On Generality” section (see Simons et al., 2017) that will include a statement with the information above about our sample (psychology students, mostly identified as women, and data loss).
The justification for the sample size is not convincing given that there are powerful tools that are very helpful in deciding on the minimum sampling size: https://github.com/moshagen/semPower/tree/master/vignettes, https://sempower.shinyapps.io/sempower/. Because data have been collected, running post hoc power analysis would seem the only sensible option. Such analysis should account for the fact that various types of invariance will be tested. Models' identification will impact the degrees of freedom for each tested model. Sensitivity analysis should provide detailed information regarding power, tested RMSEA values, and the like. (Jacek Buczny)
Response: We thank you for mentioning these tools to compute power for our analyses. As a result of this comment, we now use Sempower to calculate the sensitivity of our planned analyses (see our code via OSF: https://osf.io/mr8n3/).
We don’t think that we should only do post hoc power analyses, as we can do sensitivity analyses now already, as well as post hoc analyses after the manuscript is completed:
A priori sensitivity analyses using SemPower: We computed an a priori sensitivity power analysis for general configural models (but not specific power for metric, scalar, and residual invariance). The result of this analysis was that 164 participants would be required for the model (our previous calculation suggested 160). We believe we can do these analyses a priori, as it relies on only a limited number of assumptions:
- We computed power for a general confirmatory factor analysis (CFA) model.
- We set the amount of misfit to correspond to an RMSEA of at least .05.
- We set power at 80%.
- We set the degrees of freedom at 100.
- We set alpha at .05.
We changed the power analysis section accordingly: “We also computed power for a general configural longitudinal measurement invariance models (CFA) models. We set power to 80%, alpha to .05, the amount of misfit to correspond to an RMSEA of at least .05, and the degrees of freedom to 100. The result of this analysis was that 164 participants would be required.”(page 11).
Post hoc sensitivity analyses using semPower.powerLI: To compute the power for metric, scalar, and residual longitudinal invariance, one should specify and quantify the change in the loadings, intercepts, and residuals between measurements at Time 1 and 2. Unfortunately, we do not have such specific a priori hypotheses. Depending on how large the change would be, we may or not be sufficiently powered to detect these changes. For example, we currently have a power of 90 (with our longest subscale - 8 items) and a power of 80 (with our shorter subscale - 3 items) to detect a change from .50 to .74 in the loadings of the items (metric longitudinal invariance between T1 and T2). The R code to compute post hoc sensitivity has been included in the supplementary material on our OSF Page (https://osf.io/mr8n3/). We will run the code for each subscale of the STRAQ-1. We will also report all the steps and tests for the longitudinal invariance and their associated power in the manuscript. We will not claim to have reached longitudinal invariance for any subscales if one of the tests (metric or scalar) is underpowered, even if longitudinal measurement invariance holds based on our criteria for longitudinal measurement invariance.
Consider swapping ICC power analyses from detection of non-zero scores to estimation precision. The current power analyses for the ICCs are based on their ability to detect a non-zero ICC. Many, including - I think - Parsons, Kruijt & Fox (2019) have argued that zero is not a particularly meaningful reference point for reliability estimates. I.e., detectable non-zero reliability does not tell us much about whether reliability is practically adequate, in my opinion. I would argue that a better approach is to estimate the 95% CI width that your sample size will provide as a function of different ICC values (as CI width and estimate are related for ICC). This can be done using the R package ICC.Sample.Size. I have a working example of this here, which I've taken from an in progress test-retest study I'm running. Feel free to use or adapt this yourself: https://gist.github.com/ianhussey/03a3d3940b93d79191a3926a09bcfc2b (Ian Hussey)
Response: Thank you for recommending ICC power analyses of estimation precision and for sharing your personal code. We conducted and adjusted the power analysis section to estimate the 95% CI width that our sample will provide as a function of different ICC values (an adaptation of your code for the power analysis is available in the Supplementary Materials via our OSF page). Of course, the higher the ICC values, the more power we have to estimate the 95% CI width. Based on this power analysis, we will specify in the discussion which ICC(s) estimates are sufficiently powered to precisely estimate either their 0.2 (and 0.1 if applicable) widths of the 95% CI.
We rewrote the power analysis section accordingly and have added this sentence: “Because researchers have argued that detection of non-zero ICC scores may not be sufficient and meaningful (see for example, Parsons, Kruijt & Fox, 2019), we also conducted a power analysis to estimate the 95% CI width that our sample will provide as a function of different ICC values *. (*footnote: The R code associated with this power analysis is available in the Supplementary Materials via our OSF page: https://osf.io/mr8n3/.) This power analysis suggested that based on our sample size N = 184, we could estimate any ICC above .30 with a 0.2 width of the 95%CI, and any ICC above .80 with a 0.1 width of the 95% CI.”(page 11-12).
About Longitudinal measurement Invariance (LI)
Be more precise about your inferential method and its correspondence with Mackinnon et al. From page 13, the manuscript states that "we followed the procedure provided by Mackinnon et al. (2022)" and then goes on to specify your inferential method (e.g., which model fit indices, hypothesis tests, and cut-offs). However, most of the specifics that the current manuscript mentions are not what Mackinnon et al specify in their preprint. Indeed, my reading of Mackinnon et al is that they are purposefully non specific about the method they employ for comparisons (e.g., between configural, metric and scalar models). To take one example, Mackinnon et al. discuss the results of Cheung & Rensvold's (2002) highly cited simulation studies, which studied the utility of examining change in CFI between models ($\delta$CFI), but the current manuscript doesn't employ this method. I would also raise the point that the method the manuscript currently suggests - a combination of both the chi-square tests' p values, the CFI, and the RMSEA - has not, to my knowledge, been assessed within any of the well cited simulation studies, and therefore has an unknown sensitivity-specificity tradeoff. (Ian Hussey)
Response: We indeed plan to rely on the delta CFI to assess measurement invariance and decide which model to retain in the iterative procedure that evaluates the model fit of the configural model, metric model, scalar model and residual model for each subscale of the STRAQ-1. We now better define our criteria in the main text of the manuscript: “ Mackinnon et al. (2022) provided several criteria to access model fit for measurement invariance, one of these is the delta CFI (of .01) which is also recommended by a simulation study (Cheung & Rensvold, 2002). We decided to rely only on a ΔCFI of -.01 or more to conclude that the model with the largest CFI should be chosen. This means that if the ΔCFI is inferior or equal to -.01 we will choose the more parsimonious model and conclude for the longitudinal invariance of the specific level (metric, or scalar, or residual).” (see page 14-15). Additionally, we acknowledge in the manuscript that there is a lack of norm in the field: “ Before pre-registration, we made choices about which metrics and cut-offs we would base our conclusion and interpretation of the subscale’s performance. But we acknowledge a lack of clear norms in the field about which metric to choose for our planned analyses. So, in addition to our pre-registered metric and cut-offs, we reported the results of other fit metrics even though we did not plan to use them for inferences and did not preregister any cut-of-value for them. This process will allow other researchers, who would prefer other indicators or cut-offs than ours, to be able to evaluate our models according to their criteria.”(see page 15).
It is not clear why residual invariance will not be tested. For a helpful tutorial, see this: https://doi.org/10.15626/MP.2020.2595. (Jacek Buczny)
Response: We chose to rely on the procedure from the Mackinnon et al. (2022) tutorial, but we did not want to test for residual invariance because (1) residual invariance has been described to be hard to reach for most psychological measurement instruments (Kline, 2016; Van de Schoot et al., 2015), and (2) originally we wanted to make the ICC analysis contingent on the longitudinal invariance. But based on Ian Hussey’s comment regarding the interpretation of the measure even when the measure is not invariant (please see the section on ICC), we changed our strategy and decided not to make the ICC non-contingent on measurement invariance and we will also test the residual invariance of all the subscales of the STRAQ-1. We changed the wording about the longitudinal measurement invariance, included a description on how to test residual invariance throughout the manuscript, and we added this step in the analysis code in the supplementary materials on our OSF page: https://osf.io/mr8n3/. We will now consider the measure invariant even if longitudinal residual invariance does not hold, because even in the absence of residual invariance, scalar invariance holds. We should still be able to conclude that between T1 and T2 the constructs are the same and that the scores are comparables; ICC estimates should then indeed be interpretable.
Why did you plan to use WLS but not ML or MLR? Besides, I recommend including a script for the analysis of multivariate normality as it might be helpful in determining the correct estimator. (Jacek Buczny)
Response: In our measurement models, we decided to use the Diagonally Weighted Least Squares (WLSMV) estimator over the maximum likelihood (ML) or robust maximum likelihood. Our data is ordinal (5-point Likert type scale, see for example, Liddell & Kruschke, 2018) WLSMV is specifically designed for ordinal data. Furthermore, WLSMV makes no distributional assumptions about the multivariate normality of the data. Finally, in a simulation study, WLSMV was less biased and more accurate than MLR in estimating the factor loadings (Flora & Curran, 2004; Kline, 2016; Li, 2016).
We decided to include a test of the multivariate normality of our dataset to inform the reader about whether the data is normal or not, but – a priori – we do not expect it to follow a multivariate normality. In our analyses, this should not present a problem, as multivariate normality is not a requirement for the WLSMV estimator.
We added the following information to the manuscript: “To investigate whether the variables in our dataset followed a multivariate normal distribution, we used the function `mvnorm.etest` from the Energy package. The analysis showed that our data [does/ does not] follow a multivariate distribution (E = XX, p = .XX). A priori, we had already decided to use the WLSMV estimator instead of ML or MLR as arguments in the cfa function in lavaan to compute our CFA model, irrespective of the outcome of the test for multivariate normality. The WLSMV is the preferred solution when (a) the data is ordinal, and (b) if data is potentially not normally distributed, as it makes no distribution assumptions (see Flora and Curran, 2004; Kline, 2016; Li, 2016).”(page 13-14).
I suggest providing a better justification for why obtaining time invariant measurement would be important. Invariance is important in cross-time trait measurement because it ensures that intra-individual changes are not due to the disturbance in construct validity, regardless of when a measurement was taken. Would you expect that STRAQ-1 would capture any changes within the tested period (2021 minus 2018, so, three years)? Is that the period in which the levels of the measure constructs could even change? I do not think that the problem of within-person changes was adequately discussed in the manuscript. (Jacek Buczny)
Response: Thank you for pointing this out, we rewrote the manuscript adding a better justification about why measurement invariance is important. “In longitudinal studies, the meaning of a construct may change over time, resulting in longitudinal measurement non-invariance (Chen, 2008).”(page 13)
“The levels of longitudinal measurement invariance have different implications for the construct: (a) if the configural level holds, then the structure of the measure is similar between T1 and T2 ; (b) if the metric level hold, then the structure of the measure and the constructs are similar between T1 and T2; (c) if the scalar level hold then the structure of the measure and the constructs are similar and the mean differences between T1 and T2 can be compared. Longitudinal scalar invariance is thus the minimal level required for our planned ICC analysis that uses the means scores of T1 and T2 (Kline, 2016; Mackinnon et al., 2022).”(page 13). We also added to the manuscript an elaboration on how non-invariance could impact the ICC estimates (see our reply to Ian Hussey in the following ICC section).
Concerning the topic of within-person changes we will add this to the discussion: “According to Vergara et al. (2019), the STRAQ-1 measures was supposed to be a stable – trait – constructs that are unlikely to change rapidly in adulthood. A recent meta-analysis about personality trait development across the lifespan showed (similarly to previous meta-analysis, see Roberts & DelVecchio, 2000) that – after young adulthood – traits are indeed stable: they found the average rank-order stability to be r = .60, but with a large heterogeneity across studies (Bleidorn, et al., 2022). Nevertheless, life events (for instance, attachment traumas) are known to introduce changes in personality traits and can be linked differently to different traits (Bleidorn, et al., 2018). But because no test-retest of a scale to assess this has been conducted yet, we did not have any strong a priori hypothesis (a) about how life events could induce changes in participant responses to the STRAQ-1, and (b) about the timeframe in which such change in the measured personality traits could occur.”
About the ICCs
The manuscript notes that you will report ICCs. There are multiple variants of ICC, and multiple ways of labelling these variants. Please report which variant you intend on reporting, ideally with some justification of your choice (although the intent of the creators of the variants are not necessarily universal interpretations, making conceptual justification tricky or less objective at times), even if this imply means going with the modal ICC2. Weir (2005) "Quantifying test-retest reliability using the intraclass correlation coefficient and the SEM" provides a useful overview the different methods and labels. As I note above, it may also be useful to report more than one version. Specificity in the code and written prereg might become important here. Please also specify which code implementation you are using for these, e.g., which R library and version. (Ian Hussey)
However, it is perhaps also worth considering that (at least some forms of) ICC are argued to account for differences in means between timespoints. The current manuscript makes a hard binary re their interpretability, but depending on the ICC choice (which I return to below), these estimates may still be meaningful. Indeed, it may be useful to report multiple versions (e.g., ICC2 and ICC3) and consider their utility in light of the tests of invariance of means. (Ian Hussey)
Response: Thank you very much for this interesting suggestion. We will report and interpret ICC(2,1; which is used to evaluate absolute agreement), and ICC(3,1; which is used to evaluate consistency). Both of these ICCs are calculated through 2-way mixed models. The ICC(2,1) accounts for systematic and random error by specifying the time of measurement as a random effect in the model. The ICC(3,1) only accounts for random error because the time of measurement is not specified as a random effect in the model. We will include the code implementation in the text below, where the first three comments of this section “About the ICC” are resolved.
The current manuscript plans to report estimates of ICC only if temporal measurement invariance is found. I understand the logic of this, e.g., that the meaningfulness of the ICCs is undermined if the means are not invariant. However, it is quite likely that the scale will end up being used whether or not the means are invariant, and that readers and users might be better informed by the abstract including ICC estimates either way. I suggest that you include the ICC estimates non contingent on the results of the invariance tests, as their results will likely be relevant to future users of the scale either way. You chould instead specify that the interpretability of the ICCs is partially contingent on the the invariance. (Ian Hussey)
Response: Based on your feedback, we made the ICC analysis (and the report of the estimates) non-contingent on the results of the invariance tests. If metric longitudinal invariance is not reached for a scale(s), we will discuss the possibility that the ICCs estimate for this scale(s) may be unreliable because of a lack of longitudinal invariance.
Altogether, we indeed agree that reporting both the ICC(2,1) as well as the ICC(3,1) in conjunction with our tests of longitudinal invariance will allow us to better evaluate potential systematic differences and/or stability between time points.
To report the ICCs in the main text, we rely on norms set forth by Parsons, Kruijt, and Fox (2019): “We computed and reported ICC(2,1) to evaluate absolute agreement between the two time points and ICC(3,1) to evaluate consistency. Both of these ICCs are calculated through 2-way mixed models. ICC(2,1) accounts for systematic and random error by specifying the time of measurement as a random effect in the model. ICC(3,1) only accounts for random error because the time of measurement is not specified as a random effect in the model (Koo & Li, 2016). We estimated the STRAQ-1 subscales’ test-retest reliability between the 2-time points through intraclass correlation coefficients (ICCs) using the psych package in R (Revelle, 2018). The analysis code is available on the OSF: https://osf.io/mr8n3/.” (page 17). “For the Sensitivity subscale, the estimated agreement was .XX, 95% confidence interval (CI) = [.XX, .XX], and the estimated consistency was .XX, 95% CI = [.XX, .XX]. For the Social Thermoregulation subscale, the estimated agreement was .XX, 95% confidence interval (CI) = [.XX, .XX], and the estimated consistency was .XX, 95% CI = [.XX, .XX]. For the Solitary Thermoregulation subscale, the estimated agreement was .XX, 95% confidence interval (CI) = [.XX, .XX], and the estimated consistency was .XX, 95% CI = [.XX, .XX]. Finally, for the Risk Avoidance subscale, the estimated agreement was .XX, 95% confidence interval (CI) = [.XX, .XX], and the estimated consistency was .XX, 95% CI = [.XX, .XX].” (page 17).
We will interpret the analyses in the following ways:
If longitudinal measurement invariance does not hold (or is underpowered) and if ICC(3,1) shows a high level of consistency between the two time points, we will not claim that the subscale is reliable across time.
If longitudinal measurement invariance does not hold, the mean of T1 and T2 cannot be compared because the meaning of the constructs may have changed between T1 and T2 for participants, but if ICC(2,1) shows a high level of agreement between T1 and T2 we could show that there is a systematic (probably measurement) error between T1 and T2. In the latter case, the measure remains reliable.
If the measure is longitudinally non-invariant ICC(2,1) will allow us to further investigate whether the error is systematic or not depending on the level of agreement between T1 and T2.
In other words, a lack of longitudinal measurement invariance impacts the interpretation of the mean differences between T1 and T2, and thus impacts the interpretation of both ICC(2,1) and ICC(3,1) estimates (Mackinnon et al., 2022). We think that the impact of a lack of longitudinal measurement invariance is more problematic for the interpretation of ICC(3,1).
If measurement invariance does not hold, high reliability with ICC(3,1) could hide the fact that participants interpret the construct differently between the first time they took the survey and the second time they took it (for example, the pattern of loading of the measure would not be the same between T1 and T2, but the mean scores would be the same). We think that ICC(2,1) is less problematic because it can account for a systematic difference between T1 and T2, so if longitudinal measurement invariance holds, ICC2 can show a systematic effect between the two time points (for example, a learning effect or measurement error). In other words, if the measure is longitudinally non-invariant ICC(2,1) will allow us to further investigate whether the error is systematic, or not.
The manuscript uses cut-offs in several places, e.g., "X subscales to provide [excellent/good/moderate/poor] test-retest reliability". Please provide the cut-offs you're using and their citations, as these can be contentious, especially in light of Watson (2004) who noted that test-retest reliability studies “almost invariably conclude that their stability correlations were ‘adequate’ or ‘satisfactory’ regardless of the size of the coefficient or the length of the retest interval” (p. 326). (Ian Hussey)
Response: The cut-off values that we will use for the interpretation of the ICC result are the following : ICC values less than 0.5 will be reported as indicative of poor, values between 0.5 and 0.75 will be reported as indicative of moderate, values between 0.75 and 0.9 will be reported as indicative of good, and values greater than 0.90 will be reported as indicative of excellent reliability. At the same time, we will recognize that these cut-offs are still contentious. In our last draft, we already had the following text: “Koo and Li (2016) defined standards for the ICC with reliability being poor at ICC < 0.5; moderate at 0.5 < ICC > 0.75; good at 0.75 < ICC > 0.9; and excellent at ICC > 0.9.” We now clarified that these will be our cut-off values, by adding: “ “These are the cut-off values that we used for labeling our results. If the 95% confidence interval of an ICC estimate was in between two labels, we used both (for example, if the 95% CI interval would have been [0.83-0.94], the level of reliability would have been regarded as “good’ to “excellent”; see Koo & Li, 2016).”(page 17-18).
Following Parsons et al. (2019), we added a footnote saying that “We recognize that the discussion around cut-offs is contentious and that cut-offs are often arbitrarily chosen, which may make our values equally arbitrary (see e.g., Watson, 2004). The resulting labels (e.g., “good’) are considered as one of many means to assess the validity of a measure (Rodebaugh et al., 2016) and a first step towards defining a normative range of reliability estimates for a scale that will be applied across samples or contexts.”(page 18).
Minor points
Factor structure of the STRAQ-1 and unidimensionality of the subscales
Define methods of testing for unidimensionality. The method section includes templates for the assessment of unidimensionality, but the method and results do not define how this will be discussed. The assessment of unidimensionality, like say convergent and discriminant validity, can be a tricky and multifacetted process that is difficult to infer from a single metric. Perhaps this language needs to be toned down as well as the method explicated? E.g., stating that X metric produced Y result, which is (in)consistent with unidimensionality, while noting that this is just one metric? I'm generally arguing for both more explication of your method here, both to constrain researcher degrees of freedom and for the reader's understanding, and also some slightly more cautious language. (Ian Hussey)
What is the point of running exploratory factor analysis by means of the EMPKC() function? I do not find running this analysis necessary. Besides, N = 184 to small to perform a conclusive EFA. (Jacek Buczny)
It is not clear why each scale is going to be tested separately. By my lights, the instrument should be tested as a whole, so each test should be based on a four-factor model. I understand that due to the small sample size (N = 184), testing four unidimensional models seems the only option, but in my opinion, such a procedure is doubtful, at best. All the subscales were tested in the same survey, so they should be analyzed jointly. (Jacek Buczny)
Response: Based on both reviewers’ comments, we have decided not to report on unidimensionality, as our sample is too underpowered for a comprehensive test. The goal of the paper is not to test the factor structure of the scale as this has already been done by Vergara et al. (2019). The current goal instead is to investigate the scale’s reliability through ICC. Initially, we wanted to test unidimensionality of the subscales in our sample, only using the EMPKC() function (aiming for a 1-factor solution for each subscales) as a first extra step before running our main analysis, but we decided to remove this test and to not report it in the manuscript. We will thus not investigate the convergent and discriminant validity and not run a confirmatory factor analysis on the full STRAQ-1 model, as our test would be underpowered and thus not informative.
Nomological network of the scale
Report of the magnitudes of all correlations. Footnote 4 notes "All the reported correlations are significant, and the interested reader can refer to Vergara et al. (2019) for more details about all the correlations investigated in the original development paper." and table 1 reports some correlations as "n.s.". I think readers would be better informed by reporting all correlations (e.g., the min and max in the footnote, and the estimates in the table), and separately noting their significance or not separately. I'm not positive off the top of my head, but I think this is in line with APA guidelines, and provides useful detail for the reader's understanding. (Ian Hussey)
Response: Thank you for this comment. We now report all the correlations and their significance levels in tables in the Supplementary Materials on our OSF page: https://osf.io/86qdx. We do not report them in the running text since the number of correlations is large and is best presented using several tables.
Citation of R Packages
Please specify and cite all key R packages you use for your analyses. This doesn't have to be exhaustive, and could even be in supplementary materials, but it gives credit where credit is due, and helps with reproducibility.
Response: We agree, for transparency, reproducibility, and for credit to the authors that wrote the packages, we wrote the following section in our main text: “We used the following R packages to conduct the analysis: rio (Chan et al., 2021), janitor (Firke, 2021), tidyverse (Wickham et al., 2019), psych (Revelle, 2022), GPArotation (Coen et al., 2005), EFA.dimensions (O'Connor, 2022), lavaan (Rosseel, 2012), semPlot (Epskamp, 2022), semTools (Jorgensen, 2021), energy (Rizzo & Szekely, 2022), semPower (Moshagen, & Erdfelder, 2016), ICC.Sample.size (Zou, 2012).”. (page 11). We also added the relevant packages in our reference section.
Rewriting suggestions that we accepted
"This Registered Report provides the first measurement invariance across time points and test-retest reliability of the Social Thermoregulation, Risk Avoidance Questionnaire". Perhaps this should be "This Registered Report provides the first test of measurement invariance across time points and estimates of test-retest reliability for the Social Thermoregulation, Risk Avoidance Questionnaire"? (Ian Hussey)
Response: We changed the writing to “This Registered Report provides the first test of measurement invariance across time points and estimates of test-retest reliability for the Social Thermoregulation, Risk Avoidance Questionnaire". Thank you for pointing this out. (page 2).
"However, to date, this instrument has no test-retest reliability." Perhaps this should be "However, to date, this instrument has no estimates of test-retest reliability." (Ian Hussey)
Response: We changed the writing to "However, to date, this instrument has no estimates of test-retest reliability.” Again, thank you for pointing this out. (page 2).
Decision by Moin Syed
, posted 19 Apr 2023, validated 19 Apr 2023
Reviewed by Ian Hussey, 15 Mar 2023
Dear Olivier, Richard, Lindenberg, Caspar, and Hans,
Thank you for the opportunity to review your stage 1 RR. Test-retest reliability is a topic that I think about more and more these days, and estimates of it are unfortuantely underreported in the literature relative to its importance to substantive claims. I'm therefore glad to see you plan to provide estimates of test-retest reliability for this scale.
Please note that while the OSF project containing the code for the analyses etc is linked in the manuscript, it is currently not public. As such, I couldn't review the code.
Larger points
Contingent analysis and reporting of ICCs in the abstract
The current manuscript plans to report estimates of ICC only if temporal measurement invariance is found. I understand the logic of this, e.g., that the meaningfulness of the ICCs is undermined if the means are not invariant. However, it is quite likely that the scale will end up being used whether or not the means are invariant, and that readers and users might be better informed by the abstract including ICC estimates either way. I suggest that you include the ICC estimates non contingent on the results of the invariance tests, as their results will likely be relevant to future users of the scale either way. You chould instead specify that the interpretability of the ICCs is partially contingent on the the invariance.
However, it is perhaps also worth considering that (at least some forms of) ICC are argued to account for differences in means between timespoints. The current manuscript makes a hard binary re their interpretability, but depending on the ICC choice (which I return to below), these estimates may still be meaningful. Indeed, it may be useful to report multiple versions (e.g., ICC2 and ICC3) and consider their utility in light of the tests of invariance of means.
Report of the magnitudes of all correlations
Footnote 4 notes "All the reported correlations are significant, and the interested reader can refer to Vergara et al. (2019) for more details about all the correlations investigated in the original development paper." and table 1 reports some correlations as "n.s.". I think readers would be better informed by reporting all correlations (e.g., the min and max in the footnote, and the estimates in the table), and separately noting their significance or not separately. I'm not positive off the top of my head, but I think this is in line with APA guidelines, and provides useful detail for the reader's understanding.
Clarify choice of ICC variant
The manuscript notes that you will report ICCs. There are multiple variants of ICC, and multiple ways of labelling these variants. Please report which variant you intend on reporting, ideally with some justification of your choice (although the intent of the creators of the variants are not necessarily universal interpretations, making conceptual justification tricky or less objective at times), even if this imply means going with the modal ICC2. Weir (2005) "Quantifying test-retest reliability using the intraclass correlation coefficient and the SEM" provides a useful overview the different methods and labels. As I note above, it may also be useful to report more than one version. Specificity in the code and written prereg might become important here. Please also specify which code implementation you are using for these, e.g., which R library and version.
Consider swapping ICC power analyses from detection of non-zero scores to estimation precision
The current power analyses for the ICCs are based on their ability to detect a non-zero ICC. Many, including - I think - Parsons, Kruijt & Fox (2019) have argued that zero is not a particularly meaningful reference point for reliability estimates. I.e., detectable non-zero reliability does not tell us much about whether reliability is practically adequate, in my opinion. I would argue that a better approach is to estimate the 95% CI width that your sample size will provide as a function of different ICC values (as CI width and estimate are related for ICC). This can be done using the R package ICC.Sample.Size. I have a working example of this here, which I've taken from an in progress test-retest study I'm running. Feel free to use or adapt this yourself: https://gist.github.com/ianhussey/03a3d3940b93d79191a3926a09bcfc2b
Define methods of testing for unidimensionality
The method section includes templates for the assessment of unidimensionality, but the method and results do not define how this will be discussed. The assessment of unidimensionality, like say convergent and discriminant validity, can be a tricky and multifacetted process that is difficult to infer from a single metric. Perhaps this language needs to be toned down as well as the method explicated? E.g., stating that X metric produced Y result, which is (in)consistent with unidimensionality, while noting that this is just one metric? I'm generally arguing for both more explication of your method here, both to constrain researcher degrees of freedom and for the reader's understanding, and also some slightly more cautious language.
Be more precise about your inferential method and its corrispondence with Mackinnon et al.
From page 13, the manuscript states that "we followed the procedure provided by Mackinnon et al. (2022)" and then goes on to specify your inferential method (e.g., which model fit indices, hypothesis tests, and cut-offs). However, most of the specifics that the current manuscript mentions are not what Mackinnon et al specify in their preprint. Indeed, my reading of Mackinnon et al is that they are purposefully non specific about the method they employ for comparisons (e.g., between configural, metric and scalar models). To take one example, Mackinnon et al. discuss the results of Cheung & Rensvold's (2002) highly cited simulation studies, which studied the utility of examining change in CFI between models ($\delta$CFI), but the current manuscript doesn't employ this method. I would also raise the point that the method the manuscript currently suggests - a combination of both the chi-square tests' p values, the CFI, and the RMSEA - has not, to my knowledge, been assessed within any of the well cited simulation studies, and therefore has an unknown sensitivity-specificity tradeoff.
On the one hand, I am extremely sympathetic that you are trying to simulateously (1) create a tight preregistration and yet (2) assess (temporal) measurement invariance, for which there are few commonly agreed upon inference methods with the level of specificity in cut-offs etc required for a prereg. This creates a tricky situation for you. However, on the other hand, I think it's important to be more explicit about your choice of inference method, how well it corresponds with the method specified in Mackinnon et al (which you cite as following but arguably diverge from in some ways that have unknown importance), and indeed how much agreement there is in the field about these methods. I think it would be best - and indeed pragmatic - to include greater acknowledgement of the lack of norms in the field in this regard.
I would also suggest that you follow the recommendations of Putnick & Bornstein (2016) and report the results of other fit metrics even though you don't use them for inferences, especially given that the lack of norms for inference methods for invariance testing mean that some (or even many) readers may look at the same fit metric values and come to different conclusions.
I should qualify the above section and recommendations that I find the literature on these recommendations confusing and frequently miscited, and I myself may have gotten some of the details of what previous studies incorrect here or there. My suggestions here are well intentioned even if I get a detail wrong - My general desire is for some more detail on your methods, their sources, acknowledgement of the lack of agreement in the field, and some bullet proofing / maximising the informativeness of your results with other metrics for future readers' benefit.
Interpretation of the magnitude of test-retest reliablity
The manuscript uses cut-offs in several places, e.g., "X subscales to provide [excellent/good/moderate/poor] test-retest reliability". Please provide the cut-offs you're using and their citations, as these can be contentious, especially in light of Watson (2004) who noted that test-retest reliability studies “almost invariably conclude that their stability correlations were ‘adequate’ or ‘satisfactory’ regardless of the size of the coefficient or the length of the retest interval” (p. 326).
Interpretative guidelines
In light of Watson (2004), quoted in the previous paragraph, could the authors give some thought to preregistering how they might interpret, in their discussion, different results that they might find. Under what circumstances would the test-retest reliabilty, for example, be problematically low, or problematic for what type of analysis in what way? This is very tricky to do and not a hard requirement. However, if tricky to do before seeing the results, this is no less difficult after seeing the results. Perhaps it would be useful to then preregister what won't be claimed contingent on results, if no such contingencies can be specified ahead of time. For example, that no hard claims about the performence of the measure in terms of its reliability will be be made in the discussion. In saying this, I realise that I am raising the bar for this manuscript relative to published work. I have been thinking for a few years about how preregistration interacts with measurement work such as this, and it is certainly not a solved problem. I'm not looking to be difficult here and wouldn't hinder the publication of this work based on this point - I'm more raising the question for you (we, us, collectively) about high quality, preregistered measurement studies like this can think about how its claims are linked to its results ahead of time. Any thoughts or ideas or attempt at this would be interesting and welcome.
Smaller points
Please specify and cite all key R packages you use for your analyses. This doesn't have to be exhaustive, and could even be in supplementary materials, but it gives credit where credit is due, and helps with reproducibility.
In the abstract, you write:
1. "This Registered Report provides the first measurement invariance across time points and test-retest reliability of the Social Thermoregulation, Risk Avoidance Questionnaire". Perhaps this should be "This Registered Report provides the first test of measurement invariance across time points and estimates of test-retest reliability for the Social Thermoregulation, Risk Avoidance Questionnaire"?
2. "However, to date, this instrument has no test-retest reliability." Perhaps this should be "However, to date, this instrument has no estimates of test-retest reliability."
Reviewed by Jacek Buczny
, 18 Apr 2023
Dear Authors,
This is an interesting project. The structure of the manuscript is clear and the argumentation is concise. You may find my recommendations below.
Major Comments
(1) Data loss was substantial. Between 2018 and 2021, more than 1000 participant provided their responses, but only 184 will be included in the analyses. Data loss could be systematic, the sample of 184 may somehow deviate from the subpopulation (students), and thus, the generalizability of the results may be very limited.
(2) The justification for the sample size is not convincing given that there are powerful tools that are very helpful in deciding on the minimum sampling size: https://github.com/moshagen/semPower/tree/master/vignettes, https://sempower.shinyapps.io/sempower/. Because data have been collected, running post hoc power analysis would seem the only sensible option. Such analysis should account for the fact that various types of invariance will be tested. Models' identification will impact the degrees of freedom for each tested model. Sensitivity analysis should provide detailed information regarding power, tested RMSEA values, and the like.
(3) It is not clear why each scale is going to be tested separately. By my lights, the instrument should be tested as a whole, so each test should be based on a four-factor model. I understand that due to the small sample size (N = 184), testing four unidimensional models seems the only option, but in my opinion, such a procedure is doubtful, at best. All the subscales were tested in the same survey, so they should be analyzed jointly.
(4) Under the link https://osf.io/ab73e/, I could not find the code indicated in the manuscript. I found it here: https://osf.io/mr8n3/. The latter script seems incomplete (by the way: why a pdf not Rmd was submitted?). For instance, it is not clear what package(s) will be used in data analysis – lavaan, I presume. What is the point of running exploratory factor analysis by means of the EMPKC() function? I do not find running this analysis necessary. Besides, N = 184 to small to perform a conclusive EFA.
(5) It is not clear why residual invariance will not be tested. For a helpful tutorial, see this: https://doi.org/10.15626/MP.2020.2595.
(6) Why did you plan to use WLS but not ML or MLR? Besides, I recommend including a script for the analysis of multivariate normality as it might be helpful in determining the correct estimator.
Minor Comments
(7) I suggest providing a better justification for why obtaining time invariant measurement would be important. Invariance is important in cross-time trait measurement because it ensures that intra-individual changes are not due to the disturbance in construct validity, regardless of when a measurement was taken. Would you expect that STRAQ-1 would capture any changes within the tested period (2021 minus 2018, so, three years)? Is that the period in which the levels of the measure constructs could even change? I do not think that the problem of within-person changes was adequately discussed in the manuscript.
Kindest regards,
Jacek Buczny
Vrije Universiteit Amsterdam