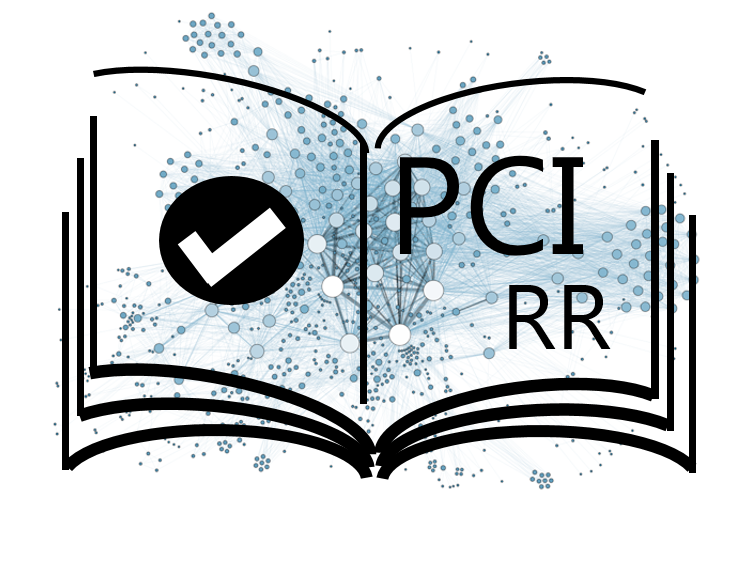
Developing differential item functioning (DIF) testing methods for use in forced-choice assessments
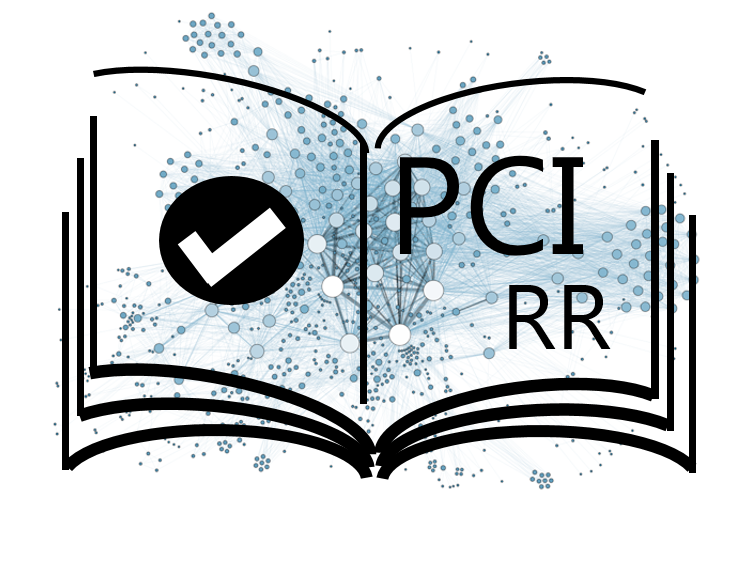
Detecting DIF in Forced-Choice Assessments: A Simulation Study Examining the Effect of Model Misspecification
Abstract
Recommendation: posted 14 February 2024, validated 14 February 2024
Montoya, A. (2024) Developing differential item functioning (DIF) testing methods for use in forced-choice assessments. Peer Community in Registered Reports, . https://rr.peercommunityin.org/PCIRegisteredReports/articles/rec?id=554
Recommendation
Level of bias control achieved: Level 6. No part of the data or evidence that will be used to answer the research question yet exists and no part will be generated until after IPA.
List of eligible PCI RR-friendly journals:
- Advances in Methods and Practices in Psychological Science
- Collabra: Psychology
- Meta-Psychology
- Peer Community Journal
- PeerJ
- Royal Society Open Science
- Studia Psychologica
References
The recommender in charge of the evaluation of the article and the reviewers declared that they have no conflict of interest (as defined in the code of conduct of PCI) with the authors or with the content of the article.
Evaluation round #2
DOI or URL of the report: https://osf.io/h6qtp?view_only=cccd50cce05a4dcea4df3a31fe963f2d
Version of the report: 2
Author's Reply, 29 Jan 2024
Decision by Amanda Montoya
, posted 29 Jan 2024, validated 29 Jan 2024
Thank you for completing your recent revision. Both reviewers indicated that your revisions addressed their concerns very well. There are a few remaining, small comments from Reviewer 1. I believe these can be addressed in a minor revision, and I do not expect to send this out again for peer review.
Reviewed by Timo Gnambs
, 22 Dec 2023
The manuscript describes a planned simulation study investigating uniform differential item functioning (DIF) in Thurstonian item response models (TIRT). I have previously commented on the manuscript and am pleased to read the revised version. I would like to thank the authors for addressing my suggestions. Therefore, I have only a few remaining comments, mainly related to some minor clarifications.
1) In Figure 2, all latent trait factor means and variances (eta) have identification constraints. Therefore, the comment in the top box may be somewhat misleading because it refers to “one” mean and variance, although all means and variances are constrained. I was wondering whether the latent means in the first-order TIRT (Figure 3) also require zero constraints on the latent means of the latent traits.
2) On Page 13, it should correctly read mu_t1, mu_t2, and mu_t3 and not t1, t2, and t3.
3) It might be helpful to readers if the introduction (e.g., in the section “Present Study”) clarified that the focus of the simulation study is on uniform DIF and that non-uniform DIF is not addressed.
4) I did not understand how the authors derived the percentages for RQ4 on Page 20. If 10% of the items in the 5-trait condition with 20 blocks and 60 items exhibit DIF, then 60 / 10 = 6 DIF items will be simulated. Since each block contains only 1 DIF item (see Table 1), there are 6 DIF blocks. This is therefore 6 / 20 = 30% and not 40% as stated in the manuscript.
5) In my opinion, simulation studies need to justify their choice of simulation conditions in order to emphasize for which real world scenarios they might be representative of. Therefore, I would hope that the authors could provide some information on the applied settings in which the chosen values of, for example, sample sizes and DIF effects are typical. Simply stating that previous simulations have used these values is not very convincing (Page 20).
Reviewed by anonymous reviewer 2, 08 Jan 2024
I have carefully examined the authors' responses to editor/reviewer comments as well as the revised manuscript. Overall, I appreciate the authors' attentiveness and responsiveness, and believe the authors have sufficiently addressed the comments and suggestions raised in the first round of reviews.
In my opinion, the revised manuscript is significantly stronger, and I look forward to the findings of the study. Therefore, I would like to express my support for the acceptance of the revised manuscript.
Evaluation round #1
DOI or URL of the report: https://osf.io/rwqba?view_only=cccd50cce05a4dcea4df3a31fe963f2d
Version of the report: 1
Author's Reply, 10 Nov 2023
Decision by Amanda Montoya
, posted 19 Oct 2023, validated 20 Oct 2023
Thank you for your submission to PCI-RR! The submitted manuscript shows promise but needs additional revision prior to further consideration. We received comments from two very engaged reviewers, and I think their comments clearly identify some steps forward that should be considered. I want to emphasize a few of their comments and provide a few of my own which I do below.
Commentary on Reviewer Comments
I agree with Reviewer 1 that incorporating non-uniform DIF into the simulation would strengthen the contribution of the study. I understand this could be a large undertaking within the study, so it may not be feasible to extend the study in this dimension. Instead perhaps it would be worthwhile to comment on whether the researchers hypothesize the results to be similar or dissimilar for uniform vs. non-uniform DIF (i.e., whether you think it's safe to generalize the results to non-uniform DIF, or whether further studies may be needed to explore this phenomenon in non-uniform DIF contexts [or mixed contexts]).
Similarly, I found the Reviewer 1's comment on sample size (equal vs. unequal) to be quite interesting. It is common to test DIF across demographic characteristics which are often unbalanced. This may have some effect on the results of the study and should potentially be incorporated or discussed. Note that Reviewer 2 suggested testing multiple sample sizes or to cite literature which suggests how sample size might impact the outcome.
I agree with Reviewer 2 that the term ipsative could be more clearly defined.
Editor Comments:
A couple things to consider because this is a registered report: Review carefully the introduction and methods as these sections will not be able to be changed after Stage 1 IPA (other than light editing). Many parts of the manuscript are written in future tense, but other parts are in past tense. I recommend revising everything to past tense because you’ll need to make these edits for Stage 2 anyway. For your simulation you should consider some positive checks that you might conduct prior to doing your analysis, that would help evaluate that the simulation has been conducted correctly. These could be any evaluation of factors that might suggest failures of the data generation or data analysis process. In simulations this might include things like, reporting rates of convergence, checking parameter bias for conditions where bias should be zero, etc.
Appendix 1. Design Table.
Please add an analysis plan column to the study design table describing how the research team will determine whether each hypothesis is supported. This could involve some test statistic or effect size, a visualization with a specific pattern, a table with a specific pattern. However, it should be clearly unambiguous how to interpret the result of the test, and not based on the judgement of the research.
In general, the interpretations column does not sufficiently account for all possible outcomes of the research results. Especially when there are multiple outcome variables and results could be mixed across these outcomes.
RQ1 should be broken into two hypotheses, as there are two outcomes. The interpretation should acknowledge the potential for contradictory results across outcomes and how such a result would be interpreted. For example, what if TIE is lower and power is also lower?
RQ2 it’s unclear how Type I Error rates will be compared. The threshold is set to .01, but is this of the estimates from each condition, or based on some kind of uncertainty estimate. In addition, it’s not clear if the second part of the interpretation is specific only to Type I Error or also power. These could be letters (a, b, or ab) to indicate which claims would be made based on the outcomes of which tests.
RQ4 This section I found very confusing. It seems that in this case a Type I Error would be when DIF is not present, but it is detected. But the interpretation suggests that if Type I Error is constant or decreases this suggests that DIF can be correctly identified, which sounds more like power than Type I error. Similar issues arise with the other interpretations, so perhaps clearly defining what is meant by a type I error in this case would be helpful.
RQ6. Please clarify what is an acceptable type I error rate and power. I found the second section of the interpretation difficult to understand.
Introduction
Equation 1 is not fully defined because the value of y is not defined if y* < 0
In Equation 2/3 is there an assumption that e_j and e_k are independent, or can they be dependent?
In general I found it difficult to follow the language of "first order" and "second order" models. This likely stems from my lack of background in this area, but I think it could be more clearly explained for interested yet less embedded audience members.
There is some discussion of the modeling distinction of DIF for an item vs. a pairwise comparison, but it was not really clear what the practical implications of this would be. Perhaps providing an example of when one vs. the other would be expected?
I found the Model Identification section to be quite difficult to read. Perhaps incorporating the notation which is introduced in the prior pages to clarify what is meant in this section would be helpful. A reviewer also suggested incorporating some of this information in a figure, which is an idea I support.
While there are obviously a lot of abbreviations used throughout the paper, I think they could be avoided in section headers to make the contents more clear to someone who might be skimming the paper.
While DIF testing by groups is somewhat of a norm, DIF can be defined and tested by continuous variables. For example, using the MNLFA approach by Bauer. It is a limitation of the study that the exploration of DIF is only by groups, and this should be acknowledged. The definition of DIF and discussion of DIF seems to imply that it only applies to groups, which is not quite right. So differentiating the kind of DIF testing this paper is doing vs. what a broader definition of DIF is.
I found the paragraph at the bottom of page 8 quite difficult to understand. I was confused because I thought the utility means had been fixed as part of the identification section, but maybe I’m not understanding which means are fixed. Similarly, the explanation of nonuniform DIF sounds backwards. Perhaps part of this is that I’m unfamiliar with using the term preferability. If preferability is the same as discrimination, would not differences on preferability correspond to uniform (rather that nonuniform DIF)? I do really struggle with the term preferability, because it seems reverse to difficulty. For example, options that many people endorse I would describe as highly preferable, but that would be low in difficulty. Again, this might be my out-of-field experience showing, but I didn’t find the language intuitive, and a cursory search did not turn up any papers using this term.
In general, I found it difficult to determine whether the IRT Models for DIF section was describing the performance of the models based on prior research, or rather whether the claims were speculative. There are very few citations in this section, suggesting perhaps there is little research in this area. But the claims seem somewhat strong. It should be more clear if these claims are merely based on the researcher’s hypotheses, or whether they are founded in prior research. Additionally, as a smaller note I found myself wondering if there are any advantages of a constrained-baseline approach. Only disadvantages were mentioned.
I also found the end of the first paragraph of page 11 to be a bit confusing. I don’t seem to understand why there is a test for the difference between the means of t1 and t2 in each group, as opposed to a test of the difference between the t1 means across groups and the difference between the t2 means across groups. This is what I was expecting but what not what was described. Perhaps this reflects a larger issue with the explanation of the setup of the model or perhaps could require some additional explanation. In the same section, I found myself feeling unclear about how exactly using the blocks accounts for multidimensionality, so perhaps this could be explained more.
Within the area of psychology that I work in, forced choice and rank order questions are not terribly popular. While I think this paper is still valuable I felt there could be more of an accounting of where these types of items are commonly used, or perhaps even more a pitch for using these types of items in contexts where they are not currently used. Perhaps prior research on these response types has identified important pockets of research communities that specifically use this kind of item and that could be described a little more in-depth.
I’m curious if the authors have any kind of hypothesis about why the free-baseline approach performed so poorly with large sample sizes in Lee et al (2021). This seems particularly relevant to the current study, as the poor performance was at the sample size selected for the current study. Was that done on purpose? Is there a rationale for why such a method would get worse with large sample sizes?
The Present Study
In general, I found the research questions to be a little difficult to understand. The wording of RQ2 is a bit awkward, I think because the question connection between the beginning and the end is lost given the length of the middle clause which summarized the result from Lee et al. 2021). In RQ3 it’s not clear what the question is asking, “more accurate” than what? RQ 5 and 6 are specific to the free-baseline approach, though it’s not clear why (as compared to the other methods). It seems like it’s likely a foregone conclusion that getting anchors wrong will harm the accuracy of the statistical models. Which I think limits how interesting RQ6 is. It seems like the underlying question, when reading the paper, might be more about whether certain methods are more robust to misspecification of anchor items than others. But that is not really how the question is framed. The latter RQs could be more specific that they are looking only at the second-order models and not the first order models.
Methods
There are still some elements of the methods which do not seem particularly clear. Specifically it’s not clear what is meant by saying that “trait correlations will be mixed across all conditions.” I initially thought this meant that trait correlations were in some way permuted (mixed) but I think what is meant is that the sign of the correlation is sometimes positive sometimes negative. The term mixed is used for so many things, I’m not sure it’s an apt descriptor on its own. Similarly, I found the information about the correlation matrix somewhat contradictory, where in one place it says that it will be matched exactly, and in other places it says it will be randomly generated.
It’s unclear from the description of the study design whether the analysis model is a within-factor (i.e., each method is used on each dataset that is analyzed) as compared to a between-factor (data generated is unique to each analysis model). My understanding that the within- method is frequently used to reduce computation time, but either would be acceptable. It should just be clear in the methods.
I do not understand Step 4 of the data generation. Measurement errors should be unique to each person, but the equation given is fixed based on lamba (a parameter that does not vary by person), so it’s not clear if this is meant to reflect the variance of the measurement errors. But as written I’m not sure this describes a process that would generate measurement errors.
Analysis Plan
Using \beta as power us somewhat unintuitive as \beta typically denotes type II errors rates, and so power is typically 1-\beta.
Reviewed by anonymous reviewer 1, 17 Oct 2023
The manuscript describes a planned simulation study investigating uniform differential item functioning (DIF) in Thurstonian item response models (T-IRT). Designed as a replication and extension of Lee et al. (2021), the authors describe several simulation conditions to evaluate the effects of, among others, anchor set size and model misspecification on DIF detection. The manuscript is well written and addresses an important topic for psychological assessments with forced-choice items. Also, the planned simulation study seems reasonable to me and likely will allow to answer the research questions. Therefore, I have a few comments that might help the authors improve their work.
1) The abstract would be informative if it emphasized that DIF was evaluated for Thurstonian IRT models that overcome the limitations of traditional scoring schemes which result in ipsative scores.
2) The figure presenting the second-order T-IRT is Figure 2 (and not Figure 1). Moreover, I believe the observed scores should be denoted by “y” and not “y*” to correspond to Equation 1. The figure would be more informative if the mean structure would be included as well. It could also be helpful to include the identification constraints described in the text, for example, the fact that 1 uniqueness and 1 utility mean per block are fixed to 1 and 0, respectively.
3) Because the authors plan to use a multiple-constraint Wald test to identify DIF, it might be informative to formally describe the respective test. I was also wondering whether the authors plan to correct the p-values for multiple testing.
4) Although the authors describe the necessary identification constraints for the single-group T-IRT, it might be informative to also describe the respective constraints in the multi-group context. I assume in the multi-group models some latent factor means and variances will be freely estimated to acknowledge between-group differences.
5) The authors plan to use Mplus for their simulation. I was wondering whether the model might also be estimated using an R package (e.g., lavaan). I am not suggesting that this would be preferable in the present situation. I was just curious whether Mplus is currently the only software to estimate these types of models.
6) The authors should adopt a consistent terminology. Currently, “Thurstonian-IRT”, “T-IRT”, and “TIRT” are used interchangeably.
7) A serious limitation of the planned study is the exclusive focus on uniform DIF. The simulation could be substantially strengthened if non-uniform DIF was evaluated as well (similar to Lee et al., 2021).
8) The authors plan to simulate two different sizes of DIF that were chosen based on previous simulation studies (see Page 17). I was wondering whether the chosen values are also representative of applied settings. Are these effect sizes common, for example, in educational assessments or other contexts?
9) The description of the simulation design does not inform about the direction of DIF. Thus, will DIF always favor one specific group or will DIF for different items favor different groups?
10) The simulation design does not specify the sample sizes of the two groups. Are the authors planning to simulate equal sample sizes in the two groups?
11) On Page 17, the authors describe how they plan to simulate DIF in the blocks. Because each block will only include 1 item with DIF (see Table 1), it might be helpful if the description emphasized that the authors plan to simulate 10% to 20% of blocks with DIF. Currently, the description focuses on items with DIF which is certainly correct but probably not what the authors want to emphasize. Moreover, the simulation conditions are inconsistently described. On Page 17, the authors report that they plan to simulate 10%, 15%, and 20% of items with DIF. In contrast, Table 2 gives values of 40%, 50%, and 60%.
12) It was unclear to me how the percentage of DIF blocks (RQ4) and misspecification (RQ6) will be implemented in the simulation. If for example, 10% of DIF blocks are simulated (RQ4) will part of these DIF blocks be included in the anchor block set (RQ6) or will additional DIF blocks be simulated specifically for the anchor block set?
Reviewed by anonymous reviewer 2, 14 Oct 2023
The current manuscript involves a simulation study that evaluates the impact of model misspecification on DIF detection. The goal of the simulation is to extend the work of Lee et al. (2021) by evaluating the performance of the second-order T-IRT model in detecting DIF under more realistic conditions (e.g., when anchors are unknown).
I thought the authors did a nice job of laying out the motivation for the paper, providing enough background, and highlighting the current gap in the literature in a compelling way. My primary concern is with where the focus is placed in regard to the interpretation of simulation results. In my opinion, the current interpretations are a bit black-and-white, and I think shifting the focus to more nuanced aspects of the results would strengthen the authors’ arguments as well as offer more prescriptive guidance to applied researchers. Below, I have included more detailed comments for refocusing interpretations along with more minor comments. The comments below are ordered based on my sense of their importance.
1. In my opinion, RQ6 (p. 31) is the most important and most interesting research question. However, Hypothesis B and the first interpretation are hard to follow (for me, anyway). First, Hypothesis B is referring to the size of the anchor set, which seems to be more in line with RQ5 (p.30). Second, for the first interpretation (if there is support for both hypotheses), the second sentence (“However, even in case of complete misspecification…”) seems to contradict the first sentence (“If we find support for both…”).
Given the importance of RQ6, I think it would be worth giving more consideration to the potential nuances that may emerge from the simulation results and dedicate an appropriate amount of space in the discussion to the interpretation of the results regarding this research question. Currently, the interpretations seem a bit limited. There is one for if both hypotheses are supported, one for if Hypothesis A is supported, and one for if Hypothesis B is supported. For this 72-cell design, there may be some interaction effects worth mentioning.
In regard to one of the potential interpretations for RQ6, the authors state that reducing model misspecification is optimal. I find this interpretation, in and of itself, to be quite uninteresting and one that most methodologists would already agree with. In line with my comments above, I think it would be more compelling to shift the focus of the interpretation to expected interactions or highlighting which specific conditions researchers should be particularly vigilant about when testing for DIF.
2. In the authors’ proposed interpretations for RQ1 (p. 29), they state that if a smaller number of traits results in improved DIF detection, this would support limiting the number of traits measured by an FC assessment. I would avoid making recommendations of this nature that encourage researchers to add or remove traits from an assessment with the goal of improving DIF detection. I think most psychometricians would agree that adding unnecessary traits or removing necessary traits would negatively impact the quality of a measure. For example, it is known that increasing the length of a test will generally improve reliability (i.e., internal consistency). However, it is not recommended to add items to a test with the goal of (artificially) improving reliability. To avoid spuriously inflating reliability, it is good practice to consult content experts or reference substantive theory, for example. I think the same logic applies to the relationship of trait size and the accuracy of DIF detection. Regardless of the simulation results, “assessments [should] be designed more intentionally only to measure what is needed rather than adding additional factors to examine DIF accurately.” This is something the authors state as a potential interpretation on p. 29, but again, I think this should always be the case. If the authors do happen to find an effect of trait size, I suggest presenting it as a consideration researchers should make when evaluating DIF as opposed to it being a motivation for altering trait size.
3. Hypothesis A and the first interpretation for RQ5 (p. 30) seem to be something that most psychometricians would already agree upon. In cases where the anchor is known to be pure (simulation studies), I think it is safe to say that a larger anchor set will always be better. Given that this set of items is used to estimate the means and SD differences between potential DIF groups, having more DIF-free items should result in better DIF detection. This has been shown to be the case for more traditional IRT models even when an anchor is not pure (e.g., Kopf et al., 2015). Given this, and similar to my comments for RQ6, I think the interpretation for the results of the research question should be refocused (e.g., potential interactions, conditions that are particularly impactful for DIF detection).
4. In the first interpretation for RQ2 (p. 29), the authors state that improved DIF detection when the DIF effect size is larger would support the use of the latent scoring approach. Although I agree that this provides further evidence for the viability of the approach, I think the study would benefit from including a comparison with at least one other model/approach. As the authors mention in the second interpretation for RQ2, findings in the opposite direction would suggest the need for a different method. Incorporating this in the current study and having results that show how the method the authors currently propose performs in relation to another DIF method would provide a useful frame of reference.
Minor comments:
5. Sample size is constant in the simulation. Although the authors provide a reasonable justification for this, I think readers will be left to wonder how much sample size could have affected DIF detection. For example, what if doubling the sample size to 2000 counteracts including DIF items in the anchor? If the authors choose not to add another sample size condition to the simulation, I would suggest providing evidence from previous work on the effect of sample size on DIF detection and/or mentioning sample size being kept constant in the limitations.
6. Although Figure 1 and the context that surrounds the use of the term “ipsative” are helpful, it might be worth providing a more explicit definition of “ipsative.” This would be especially helpful if the target audience is not likely to be familiar with FC assessments and how they are analyzed.
7. I think the path diagram labeled “Figure 1” is supposed to be labeled “Figure 2.” In addition to the figure title, there is at least one reference to this path diagram in the introduction that will need to be revised accordingly.
8. The path diagram labeled “Figure 1,” which may actually be “Figure 2,” has no caption. Including a detailed caption that allows the figure to stand on its own may be very helpful for readers, especially because it will allow readers to understand the figure without having to go back to the text (some readers may not understand the path diagram without some context).
9. On p. 5, third line from the bottom, the authors refer to “equations 2/3” in a comparison between the unit of analysis for the first-order model and the second-order model. Are Equations 2 and 3 the equations the authors meant to reference?
References
Kopf, J., Zeileis, A., & Strobl, C. (2015). A framework for anchor methods and an iterative forward approach for DIF detection. Applied Psychological Measurement, 39(2), 83-103. https://doi.org/10.1177/0146621614544195