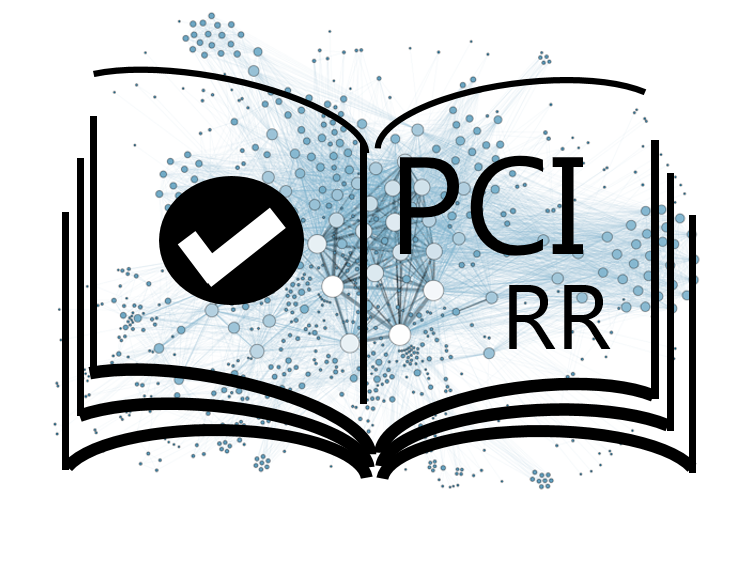
Individual differences in linguistic statistical learning and the relationship to rhythm perception
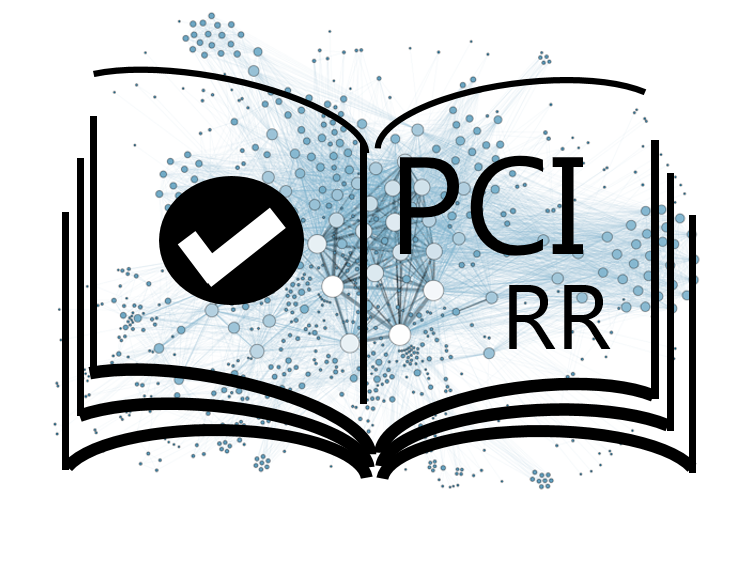
Investigating individual differences in linguistic statistical learning and their relation to rhythmic and cognitive abilities: A speech segmentation experiment with online neural tracking
Abstract
Recommendation: posted 06 December 2023, validated 06 December 2023
Wonnacott, E. (2023) Individual differences in linguistic statistical learning and the relationship to rhythm perception. Peer Community in Registered Reports, . https://rr.peercommunityin.org/PCIRegisteredReports/articles/rec?id=458
Recommendation
Level of bias control achieved: Level 6. No part of the data or evidence that will be used to answer the research question yet exists and no part will be generated until after IPA.
List of eligible PCI RR-friendly journals:
1. van der Wulp, I. M., Struiksma, M. E., Batterink, L. J., & Wijnen, F. N. K. (2023). Investigating individual differences in linguistic statistical learning and their relation to rhythmic and cognitive abilities: A speech segmentation experiment with online neural tracking. In principle acceptance of Version 4 by Peer Community in Registered Reports. https://osf.io/2y6sx
The recommender in charge of the evaluation of the article and the reviewers declared that they have no conflict of interest (as defined in the code of conduct of PCI) with the authors or with the content of the article.
Evaluation round #2
DOI or URL of the report: https://osf.io/jhbe8/?view_only=9afb8753447443d29919cc0a0b479d86
Version of the report: RR_revised_combined_27-09-2023.pdf
Author's Reply, 30 Nov 2023
Decision by Elizabeth Wonnacott
, posted 06 Nov 2023, validated 06 Nov 2023
Thank you for resubmitting your Stage 1 paper. I appreciate the very large amount of work that has gone into this revision. The two reviewers are happy with your changes, bar two very minor suggestions from one of the reviewers which you can easily implement.
I also think the paper is much improved. My only remaining concerns are in terms of the analysis plan and sample size simulations. I have spent some considerable time going through your analyses plan and script, and there are points where I don’t follow and/or where I have concerns and questions. I have listed these analysis by analysis below. Two key points I want to highlight are : (1) I question the choice (for some analyses) to use a default model representing the predictions under H1 rather than one with parameters informed by values that come out of the pilot /previous work. You justify this in terms of being more “conservative”, but in Bayes Factor terms, the use of defaults is problematic because it is likely to lead to you to finding evidence for H0 when it isn’t true (default priors generally have parameters which assume largely effects are more likely, so if the true effect is small, the data may well be more likely the null than under this H1). (See Nicenboim, B., & Vasishth, S. (2016). Statistical methods for linguistic research: Foundational ideas—part II. Linguistics and Language Compass, 10 , 591–613. https://doi.org/10.1111/lnc3.12207 and Dienes, Z. (2019). How do I know what my theory predicts? Advances in Methods and Practices in Psychological Science, 2, 364-377- and references cited therein on this point). (2) For the analyses using Bain, you need to give more details of the model of H1 and you need to have some kind of simulation that demonstrates that you will have a decent both chance of evidence for H1 if H1 is true and (critically) also for H0 if H0 is true. (NB- I previously consulted with Zoltan Dienes for his views on this type of analyses - he pointed out that a potential concern with this method is that you might not be able to find evidence for H0 if it is true, so you need to demonstrate that this isn’t the case).
I would like to ask you to address the points below in a revision. I am aware that you are under time pressure and I apologise that it has taken as long as it has to get to this stage. Note that I won’t be sending this back out to the original reviewers, however I can’t rule out that I may need to bring in someone specifically for statistical advice if I can't understand your revision / feel that evaluating it is beyond my expertise. In either case, I will do my best to turn this round for you as swiftly as I can. To speed things up, you can feel free to reach out to me personally if you have questions about the below.
COMMENTS ON THE DIFFERENT ANALYSES
Note that in the below I am assuming that all of the tests you mention are to be considered key tests of your hypotheses, and thus we need to know that, in each case, you are planning a (max) sample which give you a reasonable chance of finding evidence for H1 when H1 is true and for H0 when H0 is true. However, some may not be considered tests of critical hypotheses. For example, to replicate the key EEG results, the interaction between epoch and language condition is interesting to test for but might not be key in the way the main effect is? For such hypotheses the sample size analyses are less crucial, but then that affects what you can highlight in the abstract and focus on in your discussion. You need to clearly differentiate in that case.
Analysis for the replication of EEG results
- General: Its great that you have gone full Bayesian, but the reporting needs to reflect this. So for example in Appendix A RQ1 column 6 you talk about interpretation given “main effect of condition” and “no main effect of condition”- you should talk about this in terms of specific Bayes factor thresholds which will leave to you infer that there is evidence for H1 and (critically) also for H0. This should also be stated in the main text (also note that you shouldn’t talk about “testing significance” in the context of Bayes Factors- as you currently do on page 18). In addition, when discussing inference threshold in the main text, I would personally also make the point that, though you have thresholds for inference, since BFs are continuous you can additionally interpret them continuously (unlike p values) so the higher the BF the stronger more evidence for H1, the lower the stronger the evidence for H0. (I won’t make this point again, but in general for all of the analyses below, also go through the paper and table and remove mention of “significance”, and make sure that you are talking equally about the conclusions you will draw if you meet your criteria for concluding evidence for H1, and if you meet your criteria for concluding evidence for H0)
- lmer models:
o in the model looking for a main effect you will aim for (1+WLI|participant) which is a full random slope structure (intercepts, slope and correlation between the two); in the model also including interaction a full random slope structure would be (1+languageC*epochbundle|participant) as both WLI and epochbundle are between participants. Can you justify the choice not to have this structure? (Perhaps your simulations suggest too many convergence errors?)
o You say you will remove random slopes if you get convergence/singularity error- a small point but you could first try removing correlations between slopes – the syntax is (1+ languageC ||participant) - then if that still doesn't converge remove the slope completely (1|participant)
- Bayes factor
o The value that you set as the sd of the half normal should be a rough estimate of your expected difference between conditions. I am therefore not sure why you take the value from the previous study and divide by 2? Is it becaue you consider this to be a maximum value? Provide some explanation.
o Diennes 2020 (Dienes, Z. (2020, April 3). How to use and report Bayesian hypothesis tests. https://doi.org/10.31234/osf.io/bua5n; see also Silvey et al for a worked example) also recommends reporting robustness regions for every Bayes factor you compute. Robustness regions show the range of values of H1 you could have used and still got the same qualitative result (i.e. Bayes factor within the same thresholds as the one you report) and are a type of sensitivity analysis. (NB – this comment applies for every BF you compute, unless there is some other type of sensitivity analysis you prefer).
- Simulations
o In the table/text under the table can you more clearly say - for the sample you have finally decided to use i.e. N=105- in what % of runs you meet your threshold for inferring evidence for H1 when H1 is true (i.e. BF>6) and for inferring evidence for H0 when H0 is true, at the different thresholds of interest (BF<1/3 an BF<1/6). (NB – its important that you have explored the consequences of a higher/lower sample in your simulations- and of different thresholds- but the information in bold is what is needed to assess sensitivity of the planned sample size and this should be quick and easy for the reader to look at.)
o You have quite a lot of convergence errors/singularity errors returned. Can you maybe show that BF's are roughly the same for the set of runs where the model did/didn’t converge?
o In your Rmd file on page 23 you say that you set the value of condition to 0- I assume this is a typo and it’s the interaction that you set to 0, however I can’t check because the pdf is rendered such that it runs off the page (it needs to be reknit)
Group analysis of behavioural outcome measures
- Reader might not know the abbreviation CLMM – perhaps I missed it if not spell out cumulative link model models is
- BAIN: again, spell out acronym (Bayes informative hypotheses evaluation). More substantially, the reader needs more detail to understand how the process works. The requirements of PCRI RR state:
“For studies involving analyses with Bayes factors, the predictions of the theory must be specified so that a Bayes factor can be calculated. Authors should indicate what distribution will be used to represent the predictions of the theory and how its parameters will be specified. For example, will you use a uniform distribution up to some specified maximum, or a normal/half-normal distribution to represent a likely effect size, or a JZS/Cauchy distribution with a specified scaling constant?”
You need to do this for each BF analysis in the paper – it isn’t sufficient just to refer us to other papers for details. I am not very familiar with the Bain approach (and do please correct me if I have misunderstood) but my understanding is that for an H1 like beta1>0 the model that represents the theory is a normal (?) distribution which has a parameter for the variance which is set using a fraction of the data. Can you make these details clear including explaining how process by which the parameters are set based on the data.
- Please give details of the sensitivity analysis.
- Sample size analysis: As noted above, if this is considered a key hypothesis in the paper, you need to show that your planned sample has a reasonable chance of finding evidence for H0 if H0 is true, and for H1 if H1 is true. Is this a place where you could use the pilot data? That is, extract values from that data and generate random data from those so you can see % of samples with various N where you get evidence for H1, and then simulate data using the same parameters except changing the key parameter to 0 and seeing % of samples where you get evidence for H0?
Correlations between neural and behavioural data
- You say you use the default prior in JASP with k=1, but as per the requirements I state above, you need more explicitly spell out what distribution is used and what its parameters are. Your use of this distribution needs to be justified and, as I pointed out above, a concern in using default priors is that it is biasing you to find evidence for the null. I would suggest that values from the pilot or previous studies where you found evidence for these correlations[1] would be your best bet in terms of getting ball-park estimates of the size of correlation you expect. You can also report robustness region, or some other sensitivity analysis.
- Sample size analyse:
o Note: If I have understood your code correctly, I think that the test of whether we can find evidence for H0 if it is true is covered in section labelled H0 which is on page 25 and page 26, and the tests for when H1 is true are on page 27 - my comments relate to those
o When looking for evidence for H1/H0 it isn’t clear to me why you have focused on an analysis with a maximum of N=150, when the N you are planning to use is N=105? (Maybe the justification of sample size is based on the ASN, but if ASN=x that just tells us that on average a boundary would be crossed (and thus data collection stopped) when N=x, but it doesn’t tell us what % of runs we would correctly find evidence for H1/H0 if data collection always stopped at N=x. (Again, it’s great to have explored the implications of using different sample sizes but, as above, what we need to know to assess the planned study is the % of runs in the simulation where - using the procedure you are going to use with a particular maximum - you find evidence for H1 when it is true and H0 when H0 is true).
o Note that if you change to using informed models of H1 for each hypothesis, you will need separate analyses testing for evidence for H0 when H0 is true for each hypothesis.
Analyses of individual differences in statistical learning: Correlations
- You do some simulations looking for the possibility of detecting small, medium and large effects. However, the pattern of findings results from the fact that you are using a default model of H1, which as noted above, is likely to be testing the theory of a large effect. So you are simulating data where the effect is (e.g.) small, and then computing a Bayes Factor which tells you if this data this more likely under a theory where there is effect is large, or one where there is no effect. It isn't surprising that the answer to question is often that the null is more likely. This demonstrates why it is important to use a model of H1 that roughly matches your expectation of effect size in this scenario. Again, perhaps you can use values from the pilot/previous studies to get a ball-park estimate? And then this can be used both as a parameter in the model of H1 and in the simulations where you generate data under the assumption that H1 is true.
Analyses of individual differences in statistical learning: Mediation analysis
- You say “We will, however, only add tasks as mediators that are significantly correlated with the SSS task in the correlation analysis between all tasks above” but under the Bayesian approach you are taking you aren’t testing for significance. Do you mean that if BF >6? What if the BF doesn’t meet this criteria but also doesn’t meet criteria for accepting the null?
- Computational of Bayes Factor and sample size analyses: I got quite confused here. In the paper you say you will use Bain. As above, in that case you need to have details about how H1 is computed under that approach. But what is the relationship between using Bain and the analyses in the script? In the script, you talk about using Dienes heuristic to test the theory that there is a direct effect using the heuristic of a uniform distribution from [0, totaleffect] (which seem quite different from the Bain approach?), and then further confusingly, you actually show a simulation where you use a uniform with a distribution of [0,totaleffect=1] (whereas– as I understand it- Dienes’s heuristic was suggesting that totaleffect should be a value that can be extracted from the dataset). Finally, I am confused by why the simulations involve looking at possibility of finding evidence that SSS predicts WLI in a simple regression, not looking at the situation where there is mediation? Is there a justification for this being reasonably equivalent in terms of power?
Footnote:
[1] If you want to use pearson r value from a previous study to inform your model of H1, I am not sure how you do it in Jasp, but FYI you can use Dienes calculator as you did for the other analyses. You will have (I) your predicted r value (2) your r value from the data (3) your df of the data. You use Fisher's z transform on (1) and (2). Your model of H1 can then be a half normal with sd set to (1) and the model of the data is a has mean (2) and SE = 1/squareroot(df - 1) (see http://www.lifesci.sussex.ac.uk/home/Zoltan_Dienes/inference/Bayes.htm) I am not sure if/ how that works with the BDFA.sim() function you found though.
Reviewed by anonymous reviewer 1, 07 Oct 2023
Thank you for making the changes to the manuscript. I found the manuscript a lot clearer and had a much easier time following the different predictions. I am also glad you decided to use a continuous analysis approach for the different types of synchronizers.
I am not an expert in Bayesian analyses, but from my understanding, the sample sizes are now well-justified, and I would be happy if the study goes forward as planned.
Very minor comments:
- Figure 3 seems that it was made by taking a screenshot – as a result the nonword is underlined in red (as Microsoft Word does for “misspelled” words).
- For Figure 4, would it be possible to add a note that specifies again which test measures what (theoretical) concept? As a reader (who is not very familiar with how for example rhythm is typically assessed), it is hard to keep track of all the different abbreviations.
Reviewed by anonymous reviewer 2, 27 Oct 2023
The authors have addressed all my concerns in the revision and modified their plans appropriately, and I am therefore have no further concerns and am happy to see this accepted. I also think the switch to incremental data collection using the Bayesian analysis technique is very nice
Evaluation round #1
DOI or URL of the report: https://osf.io/d9c48?view_only=9afb8753447443d29919cc0a0b479d86
Version of the report: RR_stage1_onepdf.pdf
Author's Reply, 27 Sep 2023
The now added Supplements can be found in the OSF project, folder "Revised Version":
https://osf.io/jhbe8/?view_only=9afb8753447443d29919cc0a0b479d86
Decision by Elizabeth Wonnacott
, posted 30 Jun 2023, validated 30 Jun 2023
Thank you for submitting your work as a registered report to PCI RR. Your paper has received two reviews from experts in the area and I have also read the paper.
Both reviewers are positive, though they raise some important points about design and analyses. I agree that the paper is well written and that it potentially taps into some interesting questions. However, I concur with the reviewer concerns, and I would particularly like to emphasize and enlarge on the point made by the first reviewer about the difficulty in keeping track of your different questions in the analyses plan and – critically- the fact that your sample plan is not taking into account all of the different questions you are answering.
A requirement for being accepted as a Stage 1 RR is that for every RQ/hypothesis you are testing, you have established that you will have a sufficient sample. Every row in the big table at the end has to have its own sample size justification (NB a member of the managing board confirmed for me that this is the case). For places where you don’t want/ aren’t able to do that, you can keep those as exploratory analyses that you look at once you have the data, but then they aren’t part of your pre-registered analysis plan. This means they can’t feature in the abstract and – though you can talk about them in the discussion - they can’t be the main focus.
At present, you only seem to be powering for RQ1, which is a main effect. But your other RQ’s will have different power requirements. You need to find a way to estimate for every hypothesis you want to retain. I think that this will most likely require simulating random data.
I also would like to comment specifically on the Bayes Factors, first I think it’s great to use a method that can allow us to distinguish between evidence for H0 and ambiguous evidence, especially for the kinds of questions you are interested in (as you note, there are many papers with null effects in the literature looking at relationships between statistical learning and other factors, and it is hard to know how to evaluate without an inferential statistic that evaluates evidence for the null - Bayes Factors can avoid this problem). It would be better to be consistent be consistent in your approach though, so if using Bayes Factors for some tests, use throughout. (Or at least if you think there is there is a good reason to use in some cases and not others, this needs clear justification). Note that for Bayes Factors, when you do your sample size simulations you need to separately consider sample required for establishing H1 when H1 is true and also H0 when H0 is true (i.e. for the later, you will need to test simulated data sets where you set the parameter for the effect of interest to 0). A couple of points here: it will be much harder to find evidence for H0 being true than for H1 being true. You are very likely not going to be able to plan for 90% power of getting a chance of BF<1/6 when H0 is true. So its going to be about balancing the ideal against feasibility, but you need to show that you don’t have (e.g.) only a 20% chance of finding evidence for H0. I think it would be reasonable to use a criterion of BF<1/3 rather than BF<1/6, but this will still be challenging. I note that you plan to use the factional Bayes Factor approach- I can see the appeal as it seems to avoid having coming up with value for you predicted effect which you need for an informed model of H1. However, this may make it very hard to find evidence for H0 when it is true. You can ascertain this through simulation. An alternative is to use an informed model of H1, which uses an estimate of predicted effect size. Although I know that in some cases you don’t have values from previous work to use, there are heuristic you can apply. I strongly recommend this paper by managing board member Zoltan Dienes: Dienes Z. How Do I Know What My Theory Predicts? Advances in Methods and Practices in Psychological Science. 2019;2(4):364-377. doi:10.1177/2515245919876960) (the ratio of means heuristic might prove useful?).
While coming up with predicted effect sizes may seem challenging, note that you will in any case need some rough predicted effect size estimations in order to do power calculations (i.e. whether for frequentist or Bayesian analyses, and also whether you use power software or do it via simulation).
(NB- if you would like help with simulating data for mixed effect models I could share some scripts with you to help get started- feel free to reach out separately by email. You might also find this preprint https://psyarxiv.com/m4hju on which I am an author useful for some practical demonstrations of how you can combine linear/logistic mixed effect models run using the lmer package and Bayes Factors. An alternative – and more commonly used - is that you can use the brms package and use model comparison (this is also briefly discussed at the end of our preprint). Both approaches require you to have an estimation of your predicted effect to inform model of H1).
One other thought with regard to power: I wasn’t clear why it was necessary to do a bimodal split on the SSS task, rather than treating as a continuous variable. Might not the later been more sensitive?
So in sum, I think this is potentially a very interesting a paper, and I would love to see it as an RR at PCRI. However, you will need to find a way to show that you have resources to get an N that has a reasonable chance of giving evidence for H1 if it is true and – if using Bayes Factors– for H0 if H0 is true.
Reviewed by anonymous reviewer 1, 25 May 2023
Thank you for the opportunity to review this manuscript. I hope that my comments will be helpful for the authors to revise their experiment plan and write-up.
Introduction:
- The introduction was clearly written. I also believe that the rationale of the proposed hypotheses is clear. I like the idea of testing rhythmic/musical abilities as an underlying mechanism of statistical learning ability, and believe that the scientific questions were overall well-motivated.
- The research discussed reminded me of the “auditory scaffolding hypothesis”, which proposes that deaf and hard-of-hearing children/adults may have worse sequential statistical learning due to less access to auditory signals (though evidence for this hypothesis has been lacking in recent years). I wonder if it is worth integrating it in the introduction given it postulates a similar mechanism as the manuscript here (auditory processing impacting more general statistical learning)?
- There are a lot of different predictions at the end of the introduction, and I found it hard to keep track of all of them (and the different associated tasks). Is there a way to visually present the different components of the experiment and their predictions? A simplified version of the table that is provided at the end of the experiment may also work for this purpose. (Relatedly, I wonder if this paper is doing a bit too much by trying to investigate rhythmic/musical abilities’ contributions to statistical learning, but then also looking at working memory and vocabulary size via exploratory analyses – it might be easier to focus the manuscript if the rhythmic/musical abilities were the clear focus.)
Method:
- Please be more precise when describing some of the methodological decisions:
o Please justify power in more detail for the different DVs.
o What will count as “approximately 35 participants in each of these groups” (p. 12)? Does that mean it is ok if there are 40 people in Group 1 and 30 in Group 2? What about 45 and 25? How is this decision made?
o How exactly is the cut-off between high and low synchronizers determined? Why is this distinction needed in the first place and would an analysis with synchronizing ability as a continuous variable not be more appropriate anyway?
o What exact criteria will be used to identify bad channels? (p. 16: “Bad channels identified upon visual inspection of the data or during data collection will be interpolated”)?
o What are participant exclusion criteria? Please list these clearly. For example, it is mentioned that “data of participants can be excluded after participation in the case of technical issues that cause a premature termination of the experiment, or for failure to comply with instructions in general” (p. 12). Is it possible to pre-specify exact exclusion rules here (e.g., participants will not be included unless they followed instructions on X trials of task X)?
o What kind of data quality checks will be used?
- Will you check for hearing issues? Anecdotally, I have heard that a non-substantial percentage of students actually do not hear normally at all frequencies, but may not necessarily be aware of this. If a participant had (unknown) hearing issues, how could this affect your results?
- Why do you plan to use a forward digit span and not a backward digit span as a measure of working memory?
Other minor comments:
p. 4: “foils that were not present” – this could be easily misunderstood as foils being completely new (instead of syllable combinations that had not been present); please reword.
p. 17: I do not understand the difference between the entire exposure period and time course of exposure (“will then be calculated for each participant over the entire exposure period, as well as over the time course of exposure”). Please reword.
p. 20: How exactly are values standardized?
Not all references are in APA format (words within article titles should not be capitalized).
Consider moving the information about the pilot experiment (section 3) into a supplement. As mentioned previously, the experiment and all the resulting hypotheses are already very complex, thus making it hard to follow along exactly what was done – going back to the pilot after reading about the experiment feels disjointed.
Consider changing the title to “Investigating individual differences in auditory statistical learning and their relation to rhythmic and cognitive abilities”, given that it is unlikely that rhythmic abilities contribute to visual statistical learning (correct?).
Reviewed by anonymous reviewer 2, 26 Jun 2023
This basically looks fine to me - it's a relatively complicated behavioural and neural study looking at relationships between musical experience/ability (possibly reflecting more basic aptitude for rhythm) and statistical learning, spacifically statistical word segmentation. The hypotheses are well-motivated by the literature; the experimental protocol is quite complex but explained in sufficient detail. I did however have some concerns about the planned statistical analyses, and two question about the design. My concerns are:
1. One of the main behavioural measures of statistical learning comes from the 4-point familiarity rating that participants give to words/part words from the speech stream. However, it looks like those 4-point ratings will be analysed using a vanilla linear regression, i.e. assuming that response values from -infinity to +infinity are possible. I don't think analysing ordinal response data in this way is best practice and weird stuff can happen (e.g. the model predicting impossible negative values or values off the scale - indeed there will always be some probability mass on these impossible values in this model). I would suggest the authors either revert to the 2AFC method used in the pilot (in which case a logistic regression is fine since it is designed for 2-point response scales and never predicts impossible values) *or* if they want to continue with the 4-point response scale, it is reasonably straightforward to do an ordinal regression in R with mixed effects (I think the package ordinal has a function clmm that will do this for you) which doesn;t have the problem of vanilla linear regression on ordinal data.
2. You should include by-participant random slopes for factors which vary within-subjects - e.g. since condition (structured vs baseline) is within-subjects, any analysis which includes condition should have a random effect structure at least as complex as (1 + condition | participant). Otherwise the model is forced to assume that all participants show the same effects of condition, which can be anti-conservative (e.g. if one or two participants show a huge effect that will drag the effect of condition up in a model with no random slope; in a model with random slopes it can handle these outliers without messing up the overall estimate of the effect of condition).
3. It's not super-relevant, but in case you end up using a 2AFC approach in the main experiment: in your pilot experiment, you don't need to (and indeed shouldn't) run a t-test on %s to check if participants are above chance (same reasons as given above - t-tests don't know that %s are bounded by 0 and 100). Your logistic regression can tell you if participants are on average above chance if you look at the intercept (and code condition appropriately, e.g. using sum contrasts so that the intercept reflects the grand mean) - any intercept significantly above 0 indicates above-chance performance, since the intercept is the log-odds of a correct response and log odds of 0 = odds of 1:1, i.e. 50-50 responding.
4. Design question: Given that the structured sequence learning task always comes first, how do you rule out the alternative explanation that the difference you see between conditions in your statistical learning task is partly driven by order? Or does that confound not actually matter, given that you are interested in individual differences.
5. Design question: In the pilot it was a bit of an issue that you didn't have sufficient variability in musical expertise. Are you going to sample differently to avoid this in the main experiment, or are you hoping that a larger sample will naturally uncover more musical individuals? The worry is that if ther underlying distribution of musical ability is quite tight, a bigger sample will still have low variance here, which might mean you have quite low power to spot effects of musical ability.