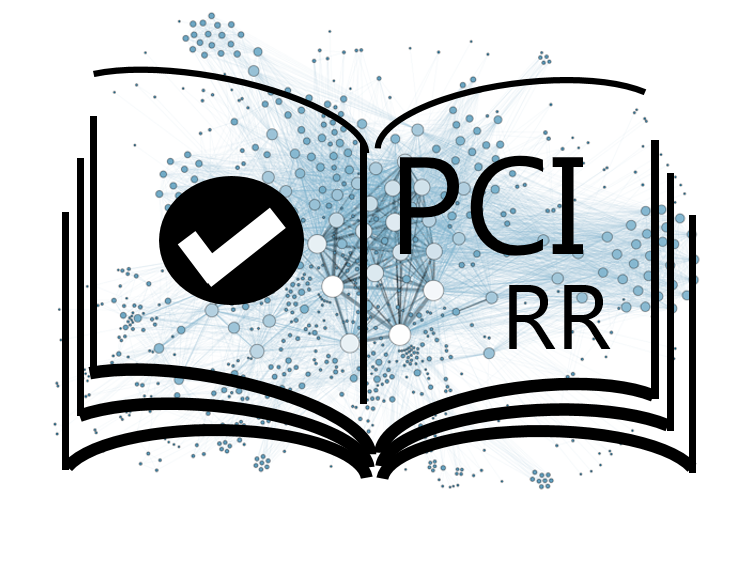
Do interim payments promote honesty in self-report? A test of the Bayesian Truth Serum
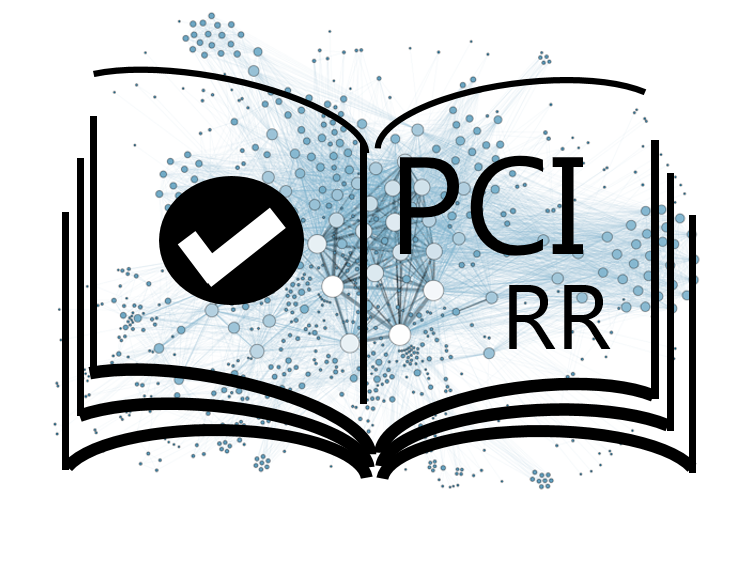
Does Truth Pay? Investigating the Effectiveness of the Bayesian Truth Serum with an Interim Payment: A Registered Report
Abstract
Recommendation: posted 27 November 2024, validated 28 November 2024
Espinosa, R. and Chambers, C. (2024) Do interim payments promote honesty in self-report? A test of the Bayesian Truth Serum. Peer Community in Registered Reports, . https://rr.peercommunityin.org/PCIRegisteredReports/articles/rec?id=781
Recommendation
List of eligible PCI-RR-friendly journals:
- Advances in Cognitive Psychology
- Advances in Methods and Practices in Psychological Science
- Collabra: Psychology
- Experimental Psychology *pending editorial consideration of disciplinary fit
- In&Vertebrates
- Meta-Psychology
- Peer Community Journal
- PeerJ
- Royal Society Open Science
- Social Psychological Bulletin
- Studia Psychologica
- Swiss Psychology Open
Truth Serum with an Interim Payment: A Registered Report. In principle acceptance of Version 3 by Peer Community in Registered Reports. https://osf.io/vuh8b
The recommender in charge of the evaluation of the article and the reviewers declared that they have no conflict of interest (as defined in the code of conduct of PCI) with the authors or with the content of the article.
Reviewed by Philipp Schoenegger, 16 Sep 2024
Dear authors,
Thank you very much for your thorough work in revising this registered report. As it stands now, the manuscript addresses most of the concerns that I outlined (and I can mostly follow your rebuttals on the points that you did not make changes, with the remnant points being minor and not worth of further delay). I especially appreciate the updated analyses and updated scales/items used in the DV. As it stands now, I am quite confident that this study will meaningfully contribute to the literature and I’m looking forward to seeing the results!
Best,
Philipp
Reviewed by Sarahanne Miranda Field
, 23 Oct 2024
Dear authors,
I have (finally, my apologies) read your revised protocol, as well as your responses to the reviewers. I am very happy with how my own suggestsions were handled (the addition of Bayesian analysis and MI in particular), and have seen that most of the other comments were also handled proactively. The ones relating to planned contrasts and one- versus two-tailed tests were, in my opinion, quite important to address.
My one comment regards this text on p. 18 of the preprint: "Bayes factors will indicate the strength of evidence for each hypothesis, with values greater than 1 supporting the corresponding hypothesis. Rather than relying on fixed thresholds, we will interpret the Bayes factors contextually following the guidance of Hoijtink et al. (2019b)." I have a few thoughts about this, and would like the authors to consider this before collecting data, perhaps even in a new, minor revision or even just in the manuscript.
- First, why talk about a threshold (in this case of 'greater than 1') if you then say that you won't rely on fixed thresholds? Second, why >1? a BF10 of 1.1 is within that range, but is practically the same as saying that both hypotheses are equally likely given the data... Why not greater than or equal to 3 (or the inverse), which is a commonly accepted threshold. Again, only if you're talking about thresholds which you claim then not to be. Do I misunderstand perhaps? If so, my apologies - it might help the reader to make this a little clearer?
- The Hoijtink article you mention in this same text passage is huge - nearly 50 pages - because it presents several options for reporting Bayesian statistics. From what you write, it's difficult to know what you might mean when you say you'll follow their guidance, because they offer a lot of guidance and for a lot of difference use cases. I suggest that because you're interested in using Bayesian inference to help contextualise the findings, it might be an idea to be specific about how Bayesian analysis can support this. For instance, by interpreting posterior probabilities which are based on the prior distribution and the likelihood of the observed data. They can be useful for parameter estimation and understanding uncertainty, which I think might be useful here (more so than a close-to-1 BF10 which isn't likely to give much information). Hoijtink's article provides much help on how to report and interpret these, so this is likely something you're already aware of but I have suggested it, just in case this is something that you hadn't considered.
I have nothing further to mention other than this. I am happy to see this be moved on in the process, and wish the authors luck with the data collection and preparation of the stage 2 RR manuscript!
I always sign my reviews,
Dr. Sarahanne M. Field
Evaluation round #1
DOI or URL of the report: https://osf.io/qhy89?view_only=9b58ae6f26434d13904e082653fee265
Version of the report: 1
Author's Reply, 01 Sep 2024
Decision by Romain Espinosa
, posted 03 Jul 2024, validated 04 Jul 2024
Dear authors,
Thank you very much for your submission. I have read your paper with great interest and received feedback from three reviewers. Given this feedback and my own reading of the paper, I recommend a major revision of your manuscript to address the concerns below.
The concerns raised in the different reports have different importance levels. I believe that some of them necessitate mandatory changes in the manuscript, while, some others, could be addressed with one or two sentences or you could explain why you think differently from the reviewers or myself.
Let me flag the most important issues I see in the reports. First, I share Philipp’ and Sarahanne's concerns about the interpretation of null results. The absence of evidence is not the evidence of absence. Here, equivalence testing or smallest-effect size of interest testing are your friends if you want to go further in this direction. Second, there is a concern about the multiple hypothesis testing that Philipp, Martin, and I felt uncomfortable with. I read Rubin (2021) that you cite and I understand your comment: you test each hypothesis separately with separate tests, so you could argue they are not part of the same family of hypotheses. However, your overall conclusion (‘overaching inferential criteria’ section) combines the results of these hypotheses. So, here, it is clear that they are part of a family of hypotheses (wrt to your conclusion). A complex question is how to address the interdependence between the tests. Third, this then raises questions about the sample size, as Martin underlines, once you have addressed the previous issue(s).
As far as I am concerned, I see no problem with sticking to a frequentist approach. Bayesian approaches can be informative in case of null results, but there are also tools in frequentist statistics. I think that the exclusion of some observations poses no problem but it might be important to think about potential issues (which I might not have anticipated). In my discipline, imputation is rarely done, so I would not push you in this direction if you do not find it appealing (except, here again, if I miss important elements).
There are other important issues raised in the reports which would at least need some clarification in the manuscript (e.g., the construction of the i-scores, etc).
Please note that I might have been mistaken in my comments (and reviewers as well) for various reasons. In this case, do not hesitate to contradict us.
I am looking forward to receiving the revised version of your manuscript.
Best regards,
Romain
——
Own comments:
Page 6: the sentence about Menapace and Raffaelli (2020) is unclear.
How do you take into account the fact that receiving an intermediary payment might convey information about whether one underestimates or overestimates other participants’ views or strategies?
Hypothesis table: I do not understand why H2 is part of the second research question. It is clear that H1 is part of RQ1, and H3 is part of RQ3. However H2 explores BTS-IP compared to no BTS, so it seems to be closer to RQ1.
It seems to me that the hypotheses are not independent. For instance, if H1 is rejected (BTS increases scores), but H2 is not rejected (BTS-IP has a positive but non-significant impact), we cannot reject H3.
If we want to compare BTS and BTS-IP, we should in BTS say that their payment will be £0.25 for Part 1, and £0.25 for Part 2, but they will know that at the end, no? (If people are risk averse, they should prefer two smaller lotteries than one large lottery to reduce risk, no?)
Why run 2-tailed tests if the predictions are directional?
Please put the fourth bullet point in « inferential criteria » in an « outcome neutral test » paragraph. I would also suggest adding some outcome-neutral tests for ceiling and floor effects given that you use a 5-point Likert scale. (Statistical power might be very small in case you have a large mass of the distribution on one of the extreme values of the Likert-scale in the control group.)
Regarding the conclusions to be drawn (« overaching inferential criteria »). For the 1st bullet point: Note that failing to reject H3 does not induce that the BTS-IP is not more efficient. As the motto says: « the absence of evidence is not the evidence of absence ». If you want to conclude on this, you should define a smallest-effect size of interest (SESOI) that you pre-register and, ex-post, see if you can reject the fact that the difference between BTS-IP and BTS is smaller than this difference. (We discuss one possibility under the name of Sequential Unilateral Hypothesis Testing, section 3, https://papers.ssrn.com/sol3/papers.cfm?abstract_id=4110803).
I think that the final decision could be illustrated with a decision tree. (See attached for an example from what I understood.)
Download recommender's annotations
Reviewed by Philipp Schoenegger, 28 Jun 2024
The Stage 1 report proposes to run an important further analysis of the Bayesian Truth Serum. Overall, the question asked is novel and interesting, irrespective of result. However, there are a number of important concerns (1-5) I would like the authors to address, as well as some smaller general issues (6 and onwards) where I invite the authors to address/clarify/add/remove/change something but where I am also happy for the authors to provide a simple explanation for their current choice instead. As such, I would like to see the authors resubmit a revised version of this report.
First, with respect to participants, is there a specific reason why the authors aim to collect responses from six nations instead of one? Prolific will easily be able to provide you with the required sample from just one country without introducing this potential (albeit unlikely) confound. Additionally, within Prolific, are you going to use further selection criteria? E.g., with respect to the number of successfully completed tasks, language competency, etc.
Second, I would ask the authors to clarify the hypotheses and the tails of their analysis. I think stating the null hypotheses (instead of the directional hypotheses) should improve clarity and also set up the authors to say more about what kind of conclusions they would draw in the case of a backfire effect (i.e., if the BTS makes responses less ‘honest’). The paper can still include directional predictions, of course, but as it currently stands there seem to be a couple of potential patterns of data that are not accounted for.
Third, I strongly suggest you adjust your p-values in a way that’s distinct from your current approach, where the adjusted level is still at 5%. I leave it up to the authors to decide an adjustment procedure that they think is appropriate, but I strongly suggest you pick a method that does not result in de facto non-adjusted p-values, as I am not convinced by Rubin (2021) and its application to your design.
Fourth, while I respect the authors’ choice in focusing on mean differences as their main outcome variable, I would suggest using at least one test of differences in response distributions as an exploratory additional analysis (that need not play into the main hypotheses but should be reported). I leave it up to the authors to pick whatever test they think is most appropriate, but I think some type of analysis may help provide a better understanding of a potential null effect.
Fifth, as far as I can tell, the authors do not provide a plan on how they deal with null effects. The preregistered outcome interpretations talk of a failure of hypothesis support (which is framed as a directional effect), so a backfire and a null effect would be treated identically, which doesn’t seem right to me. This gets back to the framing of hypothesis as directional having them stated as ‘no difference’ null hypotheses allow you to also consider scenarios where effects do not work (established, for example, by some type of equivalence tests with the bounds drawn from the effect size used for the power calculation) or actually backfire.
Sixth, you say that “the BTS will be tested for its ability to elicit truthful responses across various dimensions”. I might have missed this, but won’t you be aggregating across all question types? If so, how will you test for the effects in subsets of these questions? Will these be additional analyses? If so, these p-values also have to be adjusted and their relationship to the main hypotheses stated.
Seventh, why are you preregistering a Welch’s t-test without knowing the variances in both groups? Would it not be better to preregister the criterion by which you will decide on the test (which may well end up being a Welch’s t-test)?
Eighth, how are the questions presented to participants? Are they randomised?
Ninth, how will you include demographic variables in your analyses (if at all)?
Tenth, I would like the authors to also reflect on their choice of items, as some seem to be from work published in the 90s (and as such conducted prior to that). Is it not very plausible that some of the uncomfortable and/or sensitive topics of the 90s are quite distinct from the ones today? For example, discourses of gender and race/ethnicity have changed dramatically, and so has what is seen as sensitive, potentially putting into question your assumptions for the outcome variables.
Eleventh, some small things. I don’t think abbreviations in the abstract are necessary (page 1). Early on (page 3), you state that the BTS is ‘novel’, though I am not sure this is entirely true given the amount of work that has been done on it already. What do you mean by ‘overlapping coefficient’ (page 6)?
I look forward to seeing the revised report!
Best,
Philipp Schoenegger
Reviewed by Martin Schnuerch, 26 Jun 2024
Reviewed by Sarahanne Miranda Field
, 28 Jun 2024
Dear authors,
In general I like the idea of this study - it is an interesting topic, and seems to represent a good way of testing the BTS. I find the protocol to be clearly and thoroughly written. In particular, I appreciate the attention that has been paid to the sample size justification. There are, however, a few things I am less sure about.
1. I'm not convinced by your explanation for choosing a deletion procedure rather than multiple imputation procedure to handle missing data. While I think it's quite likely that missing data will be minimal, and that deletion is unlikely to introduce bias, why not just choose a method that is more methodologically robust? Can you explain your choice a little more, or change the strategy?
2. I'm wondering about the payment amounts - they seem so small that I am wondering if they will be enough for the purpose. They may well be, of course, but I am not convinced because you (unless I missed something) do not provide a justification for why you have chosen these amounts and why you think they will be sufficient for their purpose. Please provide a clear motivation for the amounts chosen.
3. I did not find a clear justification for 10 questions. You say that you chose this number to keep it manageable, but why do you think 10 will be manageable, and not, say 15 or 20? Have you run a pilot to actually test the time cost to participants? 10 seems to be a low number, and without sufficient motivation about why you have chosen this number, I am left wondering if that will be enough questions.
4. What will you do if you get p-values greater than your cutoff alpha? Do you just shrug and go "well, we don't really know..."? Because that's about the extent of the information you will get if you use frequentist statistics. I *strongly* recommend using a Bayesian approach along side of frequentist (or even in place of!), because it allows us to deal with pro-null evidence and because Bayes factors can provide more information value than p-values can.
Best of luck with the study going forward!
Sarahanne M. Field