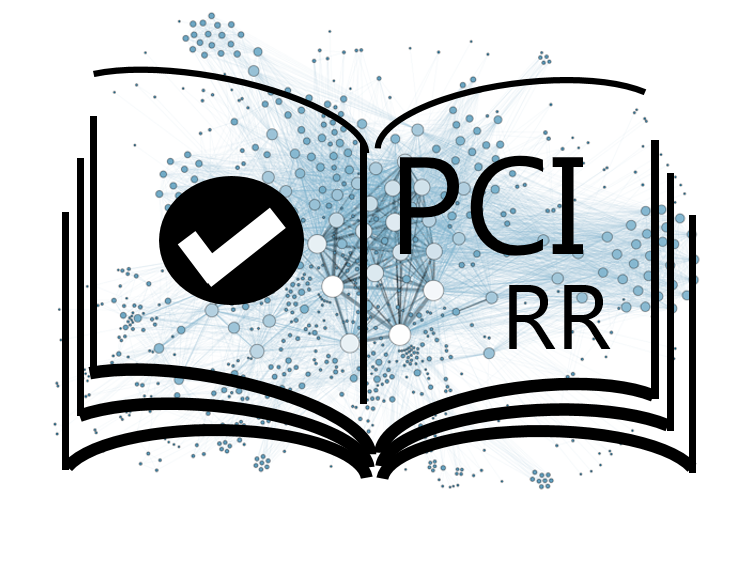
Assessing Impulsivity Measurement (UPPS-P-20-R)
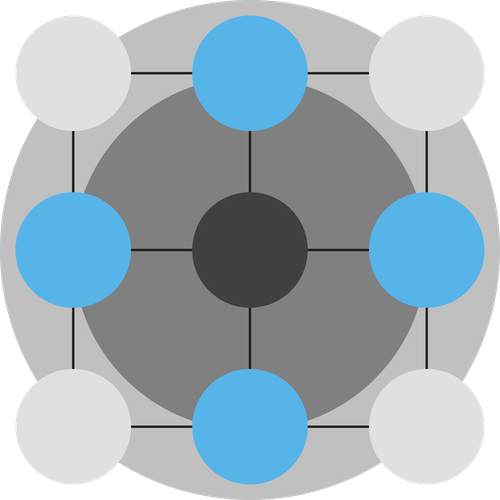
Development and evaluation of a revised 20-item short version of the UPPS-P Impulsive Behavior Scale
Abstract
Recommendation: posted 03 January 2025, validated 10 January 2025
Karhulahti, V.-M. (2025) Assessing Impulsivity Measurement (UPPS-P-20-R). Peer Community in Registered Reports, . https://rr.peercommunityin.org/PCIRegisteredReports/articles/rec?id=862
Recommendation
Level of bias control achieved: Level 6. No part of the data or evidence that will be used to answer the research question yet exists and no part will be generated until after IPA.
List of eligible PCI-RR-friendly journals:
- Collabra: Psychology
- Peer Community Journal
- PeerJ
- Personality Science *pending editorial consideration of disciplinary fit
- Royal Society Open Science
- Studia Psychologica
- Swiss Psychology Open
References
The recommender in charge of the evaluation of the article and the reviewers declared that they have no conflict of interest (as defined in the code of conduct of PCI) with the authors or with the content of the article.
Evaluation round #2
DOI or URL of the report: https://osf.io/w63hn?view_only=d6a2f8819b44492b9c3dc3caaf95be60
Version of the report: 2
Author's Reply, 20 Dec 2024
Decision by Veli-Matti Karhulahti
, posted 20 Nov 2024, validated 20 Nov 2024
Dear Loïs Fournier and colleagues,
Thank you for your patience with a small delay. One of the reviewers returned with further feedback and I think it was well worth the wait. I won’t reiterate it here but let you engage with the comments directly -- a couple of small technical follow-ups:
- The RQs certainly add clarity here. However, I suggest numbering them continuously or otherwise uniquely, which helps referring to each distinctly later (e.g., now there are three RQ1s and three RQ2s).
- I agree with the reviewer that adding at least some threshold-like criteria at this point would further add credibility later. I understand no one wants to end up with a scale development paper that concludes the scale isn’t valid and we don’t want to create a prison that leads to such conclusions with a low bar; however, spelling out some boundaries of inference also makes your inference stronger later. You don’t need criteria for each analysis, but selecting at least some key RQs and setting them e.g. gradual inference goals (X= adequate, Y= would benefit from improvement, Z= unacceptable, etc.) would be a huge epistemic jump. If it happens that some results aren’t optimal, you can always continue by exploratorily testing with alternative data/models -- it will be transparent for readers. In the end, we all want a useful scale with maximal awareness of its pros and cons. I’d love to see the field move even more away from valid/invalid semantics and be curious about different kinds and degree of utility (to be clear, I very much like it how you’re already ahead of the main curve by assessing item as well as construct level nuance; it’d be great if you can extend such sensitivity to inference too).
The idea of RRs isn’t to overly burden authors or reviewers and the manuscript is close to IPA, so I likely won’t invite reviewers for more rounds but will evaluate the final revisions myself (consulting PCI statistics if open questions remain). Therefore, please make last steps in proofreading carefully to minimize changes at Stage 2. As usual, you can contact me pre-submission if specific questions occur. Again, thanks for all detailed responses to this interesting work.
Best of health and wishes
Veli-Matti Karhulahti
Reviewed by Ivan Ropovik , 20 Nov 2024
After reading the revision of the Stage I RR, I see that the authors managed to further tighten the (already well-planned) design. Maybe a couple of outstanding issues.
# Missing data imputation vs ordinal modeling
Yes, FIML –being a ML method– cannot be implemented when you use WLSMV and treating the indicators as ordinal. So there is a tradeoff. Either you choose to use all participant’s data or use a superior method to model those. I’d do both, one as the default analysis and one as a sensitivity analysis. The question is what to go for as a default. I recommend building in a contingency for that. If the dropout due to listwise deletion (as done by lavaan) is above certain threshold, you could favor doing FIML, if it is below, you would go for WLSMV + ordinal model. The thing is that even with pretty harmless-looking absolute rate of missingness (say 5%), you can have relatively substantial double digit listwise dropout, depending on the distribution of the missingness. Then, for the research questions where the substantive interpretation would differ for these two (arbitrarily chosen) pathways, I think it would be reasonable to remain in doubt and treat the results as inconclusive.
Regarding the following sentence:
“With respect to data collection, as we will require that participants provide answers to all statements implemented in the full online survey and as we will exclude data from participants who will have failed to complete the full online survey, no missing data will arise.” (p.9)
...and later in the manuscript, the same "no missing data will arise."
It is even hard to tell how wrong this statement is. Missing data is a counterfactual phenomenon – it represents the data that would have been observed under different conditions and which inherently cannot be directly observed or retrieved but can only be estimated or inferred based on the available data and assumptions about the missingness mechanism. Some participants will be missing because of the forced responding, other data will be missing because listwise deletion will exclude a participant if they have just a single missing data point. So saying that forcing + listwise deletion = no missing data will arise is so much wrong. Getting rid of participants with nonzero missingness is not equal to having no missingness. Listwise deletion is relatively fine when the data are MCAR, but not when data are MAR or even NMAR (in that case imputation is still better than listwise deletion).
# Construct validity
In my view, the treatment of validity assessment remains weak even in the revised protocol. The authors argued here that “accounting for issues related to statistical power, a “nomological network” approach cannot be adopted”. Well, it is completely alright to consider the internal latent structure of the validated measure as the primary aim and power the study to that aim. That way, the simulations for the sample size determination need not be extended or revised. You are doing a good job with respect to the internal structure aspect of construct validity but on its own, this cannot establish substantive meaning of the underlying latent variable. This is the role of the nomological network. Currently, you are just planning to do bivariate correlations of weighted sum-scores and for the criterion/convergent constructs only resort to repetitively argue that these “present differential associations with …”. First of all, not sure what you mean by “presents differential associations”. More importantly, I reiterate my question about how the validity evidence will be assessed. Right now, there is one specific research question tied to criterion validity and one to convergent validity. But the criteria for proclaiming that the evidence speaks in favor of the given validity type are absent. Ideally, the authors would made explicit the theoretical expectations of construct interrelationships within a nomological network and test it empirically.
I understand authors’ concerns regarding the computational tractability of a model that would involve a structural model of 11 latents and 64 indicators. In my view, this can be overcome by using a two-stage estimation, specifically the Structural-after-measurement (SAM) approach (https://doi.org/10.1037/met0000503). There are ways how to model the indicators as ordinal within SAM when using lavaan, but even if you treated them as continuous (the differences with respect to substantive interpretations are usually negligible), the validation procedure would still be much more powerful. In SAM, the measurement models are being estimated separately from the structural part. The latter would thus include only 11 variables which should be feasible even with your sample size. What you gain is robustness to error propagation due to local misspecification (e.g., in some of the measurement models of the other constructs), while having all the benefits of a full SEM. This approach will allow you to see how your underlying construct functions within a far more comprehensive theoretical framework compared to an independent bunch of bivariate correlations. You could then also set some a priori thresholds taking into account the probabilistic nature of the interrelationships between the constructs, saying what you regard as good, acceptable theoretical fit, etc. (e.g., in terms of proportion of relationships within the nomological net that panned out as expected).
Overall, I think the design would benefit from having at least some straightforward minimum-threshold heuristics for saying the validity evidence is at least adequate. Now that you have included explicit RQs, I recommend going through each of those and have some sort of idea about formal way of arriving at synthesizing judgment (as many of the RQs are inherently complex). I get that you cannot have clear-cut criteria for everything in a validation but having at least minimum criteria for supporting the use of the measure would be proper to have.
# Writing
Towards the end of the manuscript, you repetitively use exactly the same phrases. Yes, we need to tighten things in an RR but a RR is still a special case of literature and that is to be read by humans (for now, at least), so I would keep that in mind.
Thank you, best wishes,
Ivan Ropovik
Evaluation round #1
DOI or URL of the report: https://osf.io/a2bm8?view_only=d6a2f8819b44492b9c3dc3caaf95be60
Version of the report: 1
Author's Reply, 18 Oct 2024
Decision by Veli-Matti Karhulahti
, posted 27 Aug 2024, validated 27 Aug 2024
Dear Loïs Fournier and colleagues,
Thank you again for submitting to PCI RR and apologies for a small delay in this Stage 1 review. Due to summer holidays and some coincidences, it took me longer than usual to find suitable reviewers and, unfortunately, one of the tentatively consenting reviewers with topic expertise had to turn down the task in the end. Considering the months that have already passed since your submission, I decided to move onwards, exceptionally with only two reviews. To compensate for the gap in construct-specific feedback, I do my best to leave related comments myself, having followed the impulsivity literature to some limited extent.
1. Although impulsivity has been a useful term for communicating certain entities related to human psychology and psychopathology, there has also been active discussion concerning its conceptual and theoretical assumptions as a construct (e.g., Zavlis & Fried 2024; see Fried 2021). I understand this goes mostly beyond scale development and I would not expect the paper to address such questions in detail, however, it would make the work stronger if the assumptions behind the chosen theory of impulsivity were more explicit. I know the authors have worked on this topic for a long time and are well aware of various conceptual and theoretical viewpoints; it would likely be a relatively small effort to address this briefly in the instruction, even though building on the existing UPPS-P work sets limits to considering it in practice.
2. As an example, I’ve personally found the conceptual distinction between ‘stopping’ and ‘waiting’ impulsivity helpful when interpreting clinical data, as in: the inability to stop using social media (after start) tends to manifest differently than the inability to resist starting (e.g., Dalley et al. 2011; Dalley & Ersche 2018). At the same time, dimensions like novelty/sensation seeking have their own literature and various parallel theories have been proposed (e.g., Mestre-Bach et al. 2020). I am noting this due to the increasing concern over multiple overlapping measures (e.g., Elson et al. 2023) -- again, your team is clearly aware of this (and addressing jingle-jangle fallacy on p. 2) but further reasoning for the superiority or utility of the chosen model would be helpful in the introduction.
3. The above could also aid further explain item exclusions/edits (pp. 6-7), which have been outlined clearly but partially lack theoretical justification. E.g., when three authors evaluated item-level content validity (p. 6), the ontology against which assessment took place is not mentioned. This becomes relevant, e.g. when modifying items to correctly measure ‘negative urgency’ (p. 7), which involves the distinction between negative/positive urgency as a premise. The rationale appears to be that assessment was done against the original 5-dimensional model, but justifying that model (over its alternatives) could make the work even more convincing. One reviewer suggests spelling out RQs for each phase; this would be an excellent opportunity to clarify the goals related to UPPS-P and impulsivity construct(s) in general.
I have not been involved in research projects explicitly on impulsivity so the comments are mainly based on my understanding of the theoretical literature; you’re naturally free to rebut any of the suggestions if they involve misunderstandings. Please also carefully consider the methodological concerns expressed by the second reviewer. I hope you find feedback useful overall and you’re naturally welcome to contact me during the process if any related questions occur. By the way, this was among the most carefully written initial Stage 1 submissions I've handled so far.
Best wishes
Veli-Matti Karhulahti
References
- Dalley, J. W., Everitt, B. J., & Robbins, T. W. (2011). Impulsivity, compulsivity, and top-down cognitive control. Neuron, 69(4), 680-694.
- Dalley, J. W., & Ersche, K. D. (2019). Neural circuitry and mechanisms of waiting impulsivity: relevance to addiction. Philosophical Transactions of the Royal Society B, 374(1766), 20180145.
- Elson, M., Hussey, I., Alsalti, T., & Arslan, R. C. (2023). Psychological measures aren’t toothbrushes. Communications Psychology, 1(1), 25.
- Fried, E. I. (2021). Lack of theory building and testing impedes progress in the factor and network literature. Psychological Inquiry, 31(4), 271-288.
- Mestre-Bach, G., Steward, T., Granero, R., Fernández-Aranda, F., Mena-Moreno, T., Vintró-Alcaraz, C., ... & Jiménez-Murcia, S. (2020). Dimensions of impulsivity in gambling disorder. Scientific Reports, 10(1), 397.
- Zavlis, O., & Fried, E. I. No evidence for a psychological trait of impulsivity. Preprinthttps://doi.org/10.31234/osf.io/m2xdj
Reviewed by Ivan Ropovik , 26 Aug 2024
Thanks to the authors for the opportunity to read their manuscript. Overall, I think that the proposed study would be informative. It is a well designed and thought-through validation study. Especially, I liked the approach to item subset selection by means of network models, which I see as conceptually strong. The authors plan to collect and examine several types of validity evidence and make them an integral part of short-form development.
That said, I have also some critical takes and suggestions for improvement. An acknowledgment upfront, I don't have much expert knowledge about the substantive aspects of the constructs measured by the present validation study. In my review, I will mainly focus on the measurement, design, and analysis side of things. As my role as a reviewer is mainly to provide critical feedback, I provide it in a form of comments below, not in order by importance but rather chronologically, as I read the paper. I leave it to the authors’ discretion which suggestions they find sensible and choose to incorporate. I hope that the authors find at least some of the suggestions below helpful.
1. I think authors well outline the risks in retaining items based on factor loadings. However, narrowing down the construct breadth is not the only risk. It is also the fact, that a “good” item among “poor” items tends to get low loading and being a naive empiricist in this sense runs the risk that the highly-correlating poor items (that measure the given construct poorly) hijack the construct validity of any scale score.
2. I suppose the each of the three exclusion criteria is sufficient for a participant exclusion. If this is the case, I suggest it is clearly stated. Also, especially given that it is a online-based data collection with limited fidelity control, it would make sense to try to screen out careless responders (e.g., based on longstring detection or some sort of insufficient variance in responding pattern, or being a multivariate outlier indicating random response pattern). E.g., the careless R package might prove useful.
3. “We will exclude data from participants who will have failed to complete the full online survey”. Why? If the responses do not show paterns of carelessness or exclusion criteria are not met, then certainly, some data is better than none. As you will be using SEM, you can leverage FIML to impute the missing data. This is conceptually a much more sound approach than using listwise deletion. Solving the missing data issue by using forcing responses comes with several –both methodological and ethical– problems. A better solution tends to be using a single reminder that the participant missed some question on the given page. Can be easily done in Qualtrics.
4. The suggested approach to handle missing data (which, according the current rules are super unlikely, as you plan to exclude anyone not finishing the survey) would turn out to be a major headache. Imagine needing to implement the entire analysis within multiple imputation approach due to, say, less than 1% of missing data. I guess literaly no one uses MI when doing latent modeling, where FIML is the natural candidate for missing data treatment.
5. The “Data” part is a rather weird structuring. I’d split information presented there into “Participants” and “Analysis” (and possibly “Sample size determination”).
6. The sample size determination is well done. You’ve arrived at a relatively tight bound between minimum sample size and the stopping rule. Maybe requiring good quality of adjustment (never heard that term though, in this context) determined by the 95th percentiles is unnecessarily strict – 90% would do the job well enough IMO – given your validation goals.
7. As you plan to use WLSMV estimator, I presume that you planned to model the latent indicators as ordinal. If this is the case (as it should be), this needs to be stated explicitly.
8. I think the primary assessment of model fit should be based on the chi^2 test, not AFIs. Chi^2 is the only formal test of model fit, and the most powerful indication of model fit. Of course, dichotomania based on whether the associated p-value is significant or not doesn’t help here. But if the chi^2 test is significant, it should be the impetus to assess local fit. Only if the there is no evidence of severe local misspecification should the model be regarded as a good-enough representation of the data. Looking solely on global fit is just not enough (regardless whether it is chi^2 or AFIs). I understand you want the RR to be as script-based as possible, but I would sacrifice some of the easiness of looking at global AFI indices and build in a procedure of assessing local fit.
9. Also, the proposed AFI cutoffs are too lenient, even Hu & Bentler (1999) suggest higher thresholds. The thing is that with your sort of (ordinal) data, model misfit is more easily to be missed, so you have to be more strict compared to situation where the data are continuous. I would go for these stricter thresholds or, alternatively, you may want to employ dynamic fit index cutoffs which is, IMO, the best option here. See McNeish, D. (2023). Dynamic fit index cutoffs for categorical factor analysis with Likert-type, ordinal, or binary responses. The American Psychologist, 78(9), 1061–1075. https://doi.org/10.1037/amp0001213.
10. “The structural equation model presenting the highest quality of adjustment to the data will correspond to the 50-item version of the UPPS-P Impulsive Behavior Scale”. How are you going to do the quality of adjustment assessment, if you have (1) model-implied standardized estimates, (2) model-implied fit indices, and (3) model-implied McDonald’s internal consistency values? I guess it cannot be purely algorithmic RR-style decision, which is fine by me. Psychometric validation isn’t easily implemented in a RR workflow.
11. I lacked more detail about how validity evidence will be assessed. I would personally consider convergent and criterion validity as part of an overwhelming concept of construct validity (aka Cronbach & Meehl notion). I think it would be a conceptually stronger solution to establish and test a nomological network, than such sort of rather piecewise evidence. But I leave that up to the author’s discretion.
Best wishes,
Ivan Ropovik
Maybe Im doing something wrong here, but the osf link doesn't work for me.
This is deliberate - it is because the final approved Stage 1 submission is temporarily privately embargoed (due to author choice). The link will become accessible once the embargo ends or is lifted.
Ah, thank you, now I also found the note!:) Maybe, there could be some kind of mark/sticker, indicating this more obvious?