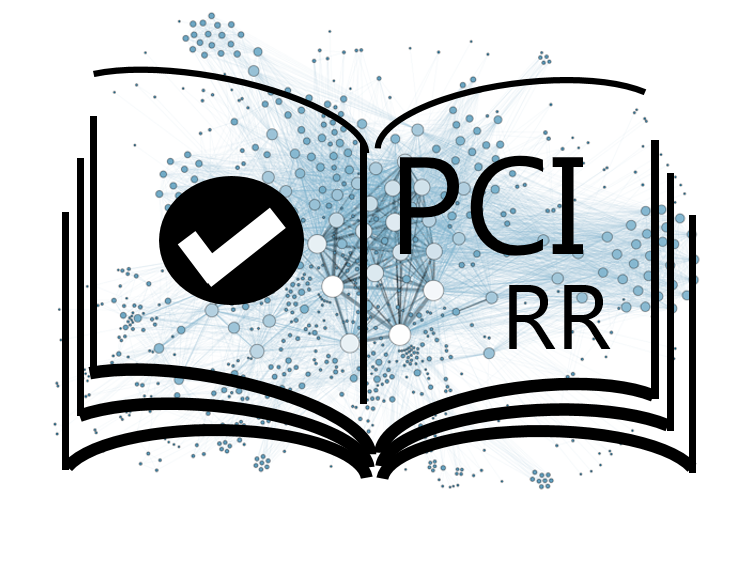
Understanding probability assessments with partitioned framing
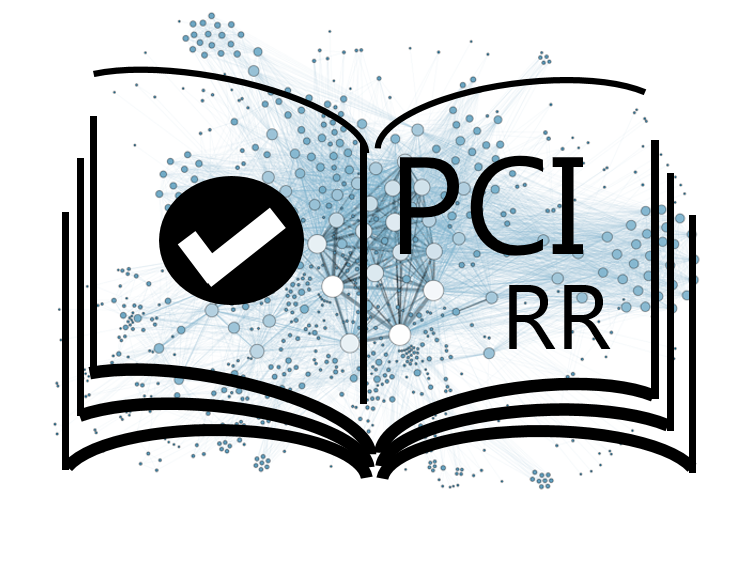
Revisiting Partition Priming in judgment under uncertainty: Replication and extension Registered Report of Fox and Rottenstreich (2003)
Abstract
Recommendation: posted 05 June 2024, validated 05 June 2024
Espinosa, R. (2024) Understanding probability assessments with partitioned framing. Peer Community in Registered Reports, . https://rr.peercommunityin.org/articles/rec?id=670
Recommendation
- Collabra: Psychology
- International Review of Social Psychology
- Journal of Cognition
- Meta-Psychology
- Peer Community Journal
- PeerJ
- Royal Society Open Science
- Social Psychological Bulletin
- Studia Psychologica
- Swiss Psychology Open
Replication and extension Registered Report of Fox and Rottenstreich (2003). In principle acceptance of Version 2 by Peer Community in Registered Reports. https://osf.io/px6vb
The recommender in charge of the evaluation of the article and the reviewers declared that they have no conflict of interest (as defined in the code of conduct of PCI) with the authors or with the content of the article.
Evaluation round #1
DOI or URL of the report: https://osf.io/gz3ad
Version of the report: 1
Author's Reply, 23 May 2024
Revised manuscript: https://osf.io/s2dvc
All revised materials uploaded to: https://osf.io/g9czs/ , updated manuscript under sub-directory "PCIRR Stage 1\PCI-RR submission following R&R"
Decision by Romain Espinosa
, posted 10 Apr 2024, validated 10 Apr 2024
Dear authors,
Thank you very much for your submission. I have read your paper with great interest and received feedback from two reviewers. Given this feedback and my own reading of the paper, I recommend a revision to address the minor concerns that the two referees raised and that I also noted while reading your manuscript.
Please note that both Olivier and I commented on the Big Ten Conference item which is, in my view, the major element of the revision. Olivier’s suggestion regarding the Bayesian statistics with the initial paper’s results used as priors can be seen as a general comment about your overall replication project and for exploratory discussions. However, I understand that you want to stick as close as possible to the tests used in the original paper. Last, both Olivier and I commented on payments. While these comments do not challenge your design, they might call for a dedicated subsection in the paper or, at least, a bit more emphasis.
I put my comments below. (As always, consider them with caution and feel free to contradict them: I might be mistaken.)
I am looking forward to receiving the revised version of your work.
Best regards,
Romain
——Recommender’s comments——
- In the original study, for Study 1, the authors have underlined some parts of the text. I checked in Qualtrics and, indeed, you have underlined these sentences. You might be willing to underline them in your paper as well (Table 5).
- You write that F&R did not mention that the Big Ten Conference had 11 teams and that they « assumed their participants would know that information ». In their paper, Note #3 discusses this point. The authors write that « most people believe the Big Ten has 10 teams, but, in fact, it has 11. ». It seems to me that the authors were aware of this uncertainty. They assumed that, overall, people would have ignorance beliefs 1/10 vs. 9/10 in the class formulation. They further add in the note: « Thus, for some participants, the class ignorance prior may have been 1/11 - 10/11. ».
Given this, you could just leave it as they did, couldn’t you? Another possibility would be, at the end of the survey, to ask a question about it. Something like: in your opinion, how many teams participate in the Big Ten Conference? (You could tailor the ignorance prior at the participant’s level.)
- I do not see any issue with the replacement of GM by IBM.
- I might be too much of an economist here but, in my view, the lack of real incentives in Study 4 is an important deviation from the original study. (People are likely to exert more cognitive efforts if they are paid, especially for significant amounts of money. 10$ here is a large amount given the time spent.) You mention this in Table 7 but not in Table 8. (By the way: you refer to Table 8 in Table 8. Is that a typo?)
- « while others were rounded to one decimal place —> 0.3 ». If I am not mistaken, they use 0.03 not 0.3.
- I think that there are some rationales for the choices of the authors of the original paper for the classification. For item 1 of S1: the chances are 1/7, which is 14.29%. So, assuming participants had to report percent integers, they could choose 14 or 15. So the authors considered both answers as correct. For the sports items: the probability is 1/10 (=10%) if they believe that there are 10 teams and 1/11 (=9.09%) if they think that there are eleven teams. So, they considered both answers as correct. For item 3, I agree that they should have considered 3% and 4% if they used the same method as for item 1. For Study 3, they can have used the same rule as for item 2 from S1 (in the case people thought there were 10 teams).
—> In my opinion, the original approach is very strict in determining which answers fit the class partition beliefs. People are not very good when it comes to probabilities. I think that your approach is more appropriate in this regard. Personally, I would have preferred a more continuous measure of the closeness/distance to one theory relative to the other. But this would bring you too far from the replication. (Maybe as exploratory discussions?)
Reviewed by Olivier L'Haridon, 29 Mar 2024
The replication study is carefully designed and planned; I have only minor comments.
My first comment refers to Study 1a, Item 2. Due to the changes in the league, the ignorance prior is now 1/7, as explained by the authors. My point here is that the league was chosen because it included a genuine ignorance prior of 1/10. An alternative for the replication would be to use another league with a genuine prior of 1/10 instead of replicating the design with the initial league but changing the prior.
My second comment refers to the payments. One dollar in 2002 is equivalent to approximately 1.65 dollars in 2024. From the replication design, I understand that payments will be anchored on the minimum wage per hour. The authors should provide a better justification for not simply replicating the initial payments, adjusted for inflation (or some other purchasing power parity index).
My last comment is a simple suggestion on statistical methods. An additional statistical method, if relevant in the current context, would be to use Bayesian statistics (e.g., Bayes factors) with the initial paper used as a prior.
Reviewed by Don Moore, 30 Mar 2024
Download the review