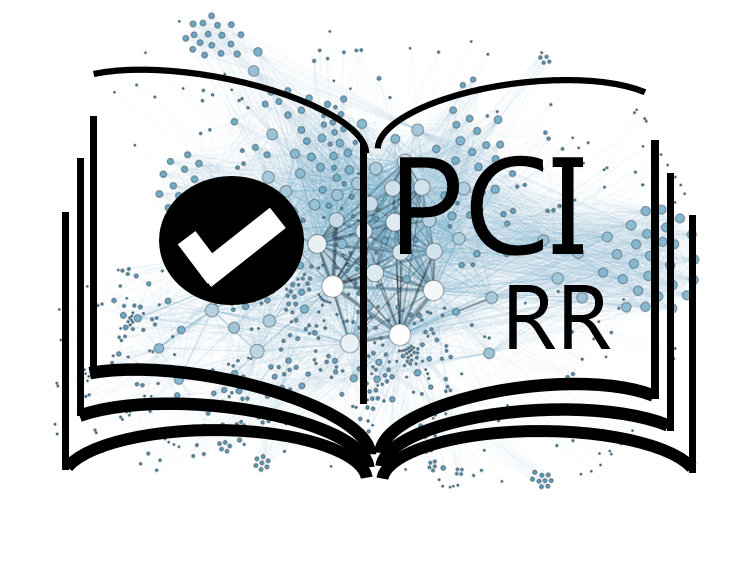
Understanding how object-oriented emotional attachment influences economic response to loss
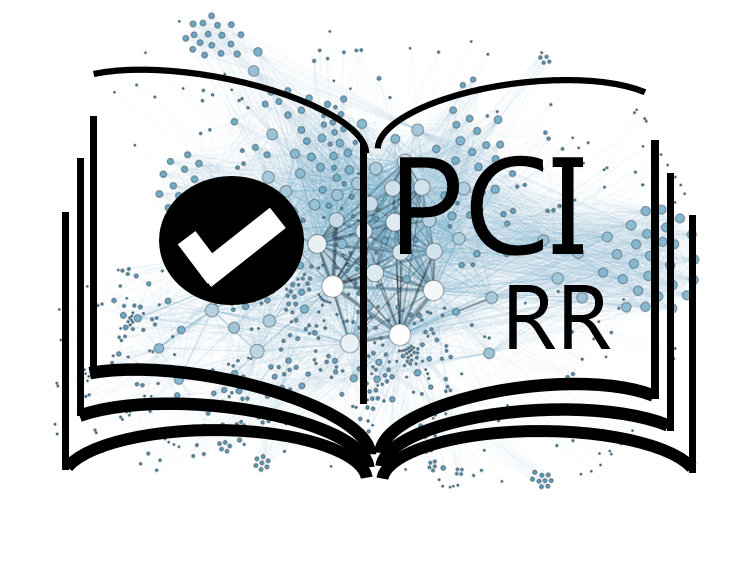
Revisiting the impact of affection on insurance purchase and claim decision-making: Replication and extensions Registered Report of Hsee and Kunreuther (2000)
Abstract
Recommendation: posted 15 June 2023, validated 15 June 2023
Chambers, C. (2023) Understanding how object-oriented emotional attachment influences economic response to loss. Peer Community in Registered Reports, . https://rr.peercommunityin.org/articles/rec?id=395
Recommendation
Level of bias control achieved: Level 6. No part of the data or evidence that will be used to answer the research question yet exists and no part will be generated until after IPA.
List of eligible PCI RR-friendly journals:
- Advances in Cognitive Psychology
- Experimental Psychology
- F1000Research
- Journal of Cognition
- Meta-Psychology
- Peer Community Journal
- PeerJ
- Royal Society Open Science
- Swiss Psychology Open
2. Law, Y. Y. & Feldman, G. (2023). Revisiting the impact of affection on insurance purchase and claim decision-making: Replication and extensions Registered Report of Hsee and Kunreuther (2000), in principle acceptance of Version 3 by Peer Community in Registered Reports. https://osf.io/b7y5z
The recommender in charge of the evaluation of the article and the reviewers declared that they have no conflict of interest (as defined in the code of conduct of PCI) with the authors or with the content of the article.
Evaluation round #2
DOI or URL of the report: https://osf.io/csabh
Version of the report: 2
Author's Reply, 10 Jun 2023
Revised manuscript: https://osf.io/ye864
All revised materials uploaded to: https://osf.io/ad6xj/, updated manuscript under sub-directory "PCIRR Stage 1\PCI-RR submission following R&R"
Decision by Chris Chambers
, posted 08 Jun 2023, validated 08 Jun 2023
I have now received two re-reviews. There is just one remaining issue to clarify concerning the distribution of the prior (and whether an informed prior may be more statistically sensitive). Please consider this point in a final revision and response, and we will then be ready to move forward with Stage 1 IPA.
Reviewed by Rima-Maria Rahal
, 30 May 2023
I have read the carefully argued responses of the author team to concerns raised, believe that these concerns are sufficiently addressed, and have no further comments. I'm interested to see the results of the replication, and wish the authors much success with implementing the study.
Reviewed by Bence Palfi, 07 Jun 2023
I thank the authors for revising the manuscript and for thoroughly addressing the comments of the reviewers. I only have a minor comment regarding the specification of the prior of the Bayes factor. Otherwise, I`m happy for the project to proceed and I`m looking forward to seeing the Stage 2 submission soon.
I presume that the Bayesian analysis will use a Cauchy distribution to model the predictions of H1. This is a heavy-tailed distribution which assumes a wide range of effect sizes to be plausible under H1, especially if the mode is large like the chosen value of 0.7. This means that the Bayes factor will have a strong bias towards evidence for the null when the real effect size is small. For this reason, I would recommend reducing the mode of the distribution. For instance, you could use the same effect size that is utilised for the sample size estimation (0.330).
Evaluation round #1
DOI or URL of the report: https://osf.io/bqwmn
Version of the report: 1
Author's Reply, 29 May 2023
Revised manuscript: https://osf.io/csabh
All revised materials uploaded to: https://osf.io/ad6xj/, updated manuscript under sub-directory "PCIRR Stage 1\PCI-RR submission following R&R"
Decision by Chris Chambers
, posted 25 Apr 2023, validated 25 Apr 2023
I have now received three reviews of your Stage 1 submission. Overall, the reviews are encouraging and suggest that the manuscript will be suitable for Stage 1 IPA following a careful and comprehensive round of revision. The main issues raised by the reviewers are clarification of conceptual terms and a number of design considerations, including potential effects of demand characteristics, inclusion of positive controls/outcome neutral tests, adequacy of both the statistical sampling plan and inferential analysis plan, and the combination of multiple studies in a single unified data collection. All of the issues raised fall within the normal scope of a Stage 1 evaluation, so I am happy to invite a revision and response.
Reviewed by Rima-Maria Rahal
, 12 Apr 2023
Law & Feldman propose a replication and extension of Hsee & Kunreuther (2000), to test if affection towards an item boost insurance purchasing and claim decision-making. The outlined hypotheses are testable, and will speak clearly to the claim that affect towards goods matters for insurance decisions.
Materials:
I found the submitted materials well prepared and very thorough, so that further replications based on these materials would be possible. Decisions about handling the materials are clearly outlined. Nevertheless, two things stood out to me that I would find debatable: First, I would take issue with the decision to remove the sentence “The whole process will take 4 hours.” from the stimulus materials. I would guess that this sentence was included in the original materials to obtain tighter experimental control over participants’ beliefs of how cumbersome the process of filing an insurance claim would be. As such, this sentence would be helpful to reduce noise in the data (e.g., some participants estimating that they would have to drive for hours to reach the office of the company, while others assuming that the office is right around the corner). Second, I am unsure about the four-in-one approach, where participants are shown all four sub-studies within subjects. It seems to me like it would be easy to guess the treatment variations at play, which may weaken the interpretability of the results. This is particularly the case because the decisions are hypothetical (as in the original paper, so this point itself is not a criticism of the replication attempt). A more conservative approach would be to present the materials as one-shot decisions, or to include a follow-up analysis that tests if there are differences in the effects elicited from the first decision and from the subsequent decisions where treatment variations may have become apparent.
Sampling and Data Collection:
An a priori power analysis is included, and generous upward correction provides confidence that the study will be well powered. It is clear that no data has been collected yet, and that data from 30 participants will be obtained to pretest the duration of the study to adjust payments. Extensive safeguards of data quality are included. I cannot foresee any ethical risks from this data collection.
Data Analyses and Potential Results:
The data analysis strategy is well prepared. However, possible interpretations given different outcomes should be stated more explicitly. This applies to both the individual hypothesis tests, where it should be clear which specific outcomes will confirm the hypotheses, and the overall evaluation of the replication attempts. I understand that the comprehensive method outlined in LeBel et al. (2018) will be used, but the registered report should be updated to clearly reflect how the specific outcomes of the replication attempts will be interpreted.
Reviewed by Fausto Gonzalez, 18 Apr 2023
The authors propose a replication of studies 1, 2, 4, and 5 from Hsee & Kunreuther (2000). They will perform a direct replication and some extensions.
The proposed replication plan is generally sound, and the replication (as opposed to extension) versions of the materials match the original studies. If possible, the authors should increase the overall sample size. I worry the “250 per each of the four conditions” criteria may lead to underpowered studies. I acknowledge that the authors are using the effect size estimates from the original studies as a basis for their sample size. Still, the small sample sizes of the original studies may not reflect the true effect size range. Another suggestion in the data collection phase is to add that MTurk participants meet a 95%+ HIT approval rate. Otherwise, the replication attempt looks to be in a good state. One small thing to note is that on pages 8 and 28 of the materials pdf on the OSF page, where the high affection and low affection camera scenarios are meant to be, it incorrectly repeats the painting scenario. This should be corrected on the OSF page (and likely on the survey since the pdf is an exported version of the survey).
Reviewed by Bence Palfi, 24 Apr 2023
The manuscript aims to replicate an established phenomenon according to which emotional attachment to an object is related to insurance decisions about the object. I believe that the proposed registered replication report is relevant and very promising. It would certainly be intriguing to see if this influential effect replicates. I applaud the authors for choosing the RR format and for the level of transparency and rigour regarding their design, materials and data collection plan. However, I have identified some issues regarding the clarity of the concept of interest, the design and the planned analyses (or lack thereof) that I believe should be addressed before the in-principle acceptance is secured.
Critical issues
I like the authors` approach to focus on the replication of a main effect first, and only investigate the topic further if the main effect is established. I`ve found the introduction convincing and clear about the justification of the project, but I think the introduction lacks some clarity regarding the investigated concept (affect) and some consideration of the potential underlying mechanisms of the main effect. Defining and clarifying the concept would be crucial so that the readers can assess the validity of the hypotheses and the materials. While reading the manuscript it was unclear to me what exactly is meant by high vs low affection and how it can be evoked/observed. It is great that all materials are transparently reported in the appendix, however, I feel that the high vs low affect manipulations are so critical to this project that they should be reported in the main text. Also, the 4 scenarios have quite different ways to manipulate high/low affection. I think it would be ideal if some explanation or description were added about how and why these interventions were used in the original paper.
The role of demand characteristics. I understand that this is a replication attempt of a phenomenon, but I believe that understanding why the effect appears is also important. Hence, I would like to invite the authors to consider the impact of demand characteristics in the current design. When reading the materials, I had the impression that explicitly telling people how they should emotionally evaluate a specific object is suggestive of the experimenter`s expectations and may give away what the affection hypothesis is about. For instance, the participants are told that they should think about an object as being very important to them, and then they are given the opportunity to demonstrate this expected commitment by reporting that they would drive as much as it takes to claim insurance (even if in real life they would not do so). Using a between-groups design reduces the impact of demand characteristics but I think it is still plausible that the main effect is driven at least to some extent by compliance.
Lack of outcome-neutral tests. One of the key features of RRs is the existence of outcome-neutral tests to ensure that the collected data are good enough to test the main question of interest. This is related to the ability of the IV to evoke the intended changes (high vs low affection) and to the ability of the DVs to pick up on the differences between the conditions. First, clarifying what exactly is meant by “emotional attachment” is important so that the readers can assess the validity of the intervention. For instance, if emotional attachment is related to feeling sad after losing the object, then you would expect people to report being sadder after losing a high affect than a low-affect object. This could be tested with an additional question, but this may not be necessary. Second, regarding the DVs: some outcome-neutral tests probing floor and ceiling effects, and the sensitivity of the DVs should be applied. For instance, the maximum amount of payment/ number of hours of driving measures have artificial upper bounds so they may have ceiling effects. By sensitivity, I mean the ability of the DV to detect a difference between the conditions. For instance, this could be tested by checking if objectively different insurance claims ($200 vs £100) evoke different responses: the vase in study 4 is worth $200 whereas the rest of the items are worth $100. Do people respond in the baseline (low affect) conditions differently for the vase than for the rest of the items? If not, then the DV may not be sensitive enough to detect the impact of the affection manipulation either.
Analysis plan. I`m not sure why is there a need to run post-hocs when it is a 2x2 design? The main effect (high vs low affect) already tests the key question of the study. This test is analogous to an independent t-test simply comparing high vs low affect groups.
I agree with the authors that the standardisation in terms of what DVs are used throughout the study is a good idea. It is better to use the same measure throughout all situations. However, using all the measures from the original study introduces the problem of measuring one concept with multiple items (likelihood and payment/driving variables). Do I understand correctly that the authors will always use the same version (either the likelihood or the payment/driving) of the variable to test the main question that was used in the original article? If so, how will the authors interpret the alternative variables, especially if there is a conflict between the findings of the two versions? I think this issue relates to the problems raised by multiverse analyses, and how different operationalisation can lead to divergent results. I believe that it would be ideal to commit to one version of the DV for each Study/situation and check the other version as a robustness test only, and raise this issue in the discussion section (especially if there is some conflict between the DVs).
I think there is also a high level of heterogeneity across the interventions. Different scenarios have very different high affect interventions so it can easily happen that they produce conflicting results. How will these findings be interpreted?
Statistical inferences. The authors may find it difficult to interpret some of their results given that they are non-significant. I recommend the inclusion of Bayesian analyses (the Bayes factor) so that the authors can distinguish between inconclusive results and clear evidence for the null. I think this is especially important for replications where the predictions of the alternative hypotheses can be easily determined based on the original study (e.g., you can use the original effect size or a discounted version of it, such as 2/3 of the original effect size), and it feels important to be able to distinguish data insensitivity from true null findings (for an example, see Bago et el., 2022 in Nature Human Behaviour). Bayes factors can be included conditionally (in case a test is non-significant) or they can be run for every single statistical test. JASP (https://jasp-stats.org/) offers a simple way to run Bayesian ANOVAs and t-tests.
Minor issues
- Parts of the words in Figure 2 are missing in my version.
- The first funnelling question (“What do you think the purpose of the last part was?”) is unclear to me: what do you mean by “last part” here? Also, how will you use responses to this question? Will you exclude participants based on their responses? I