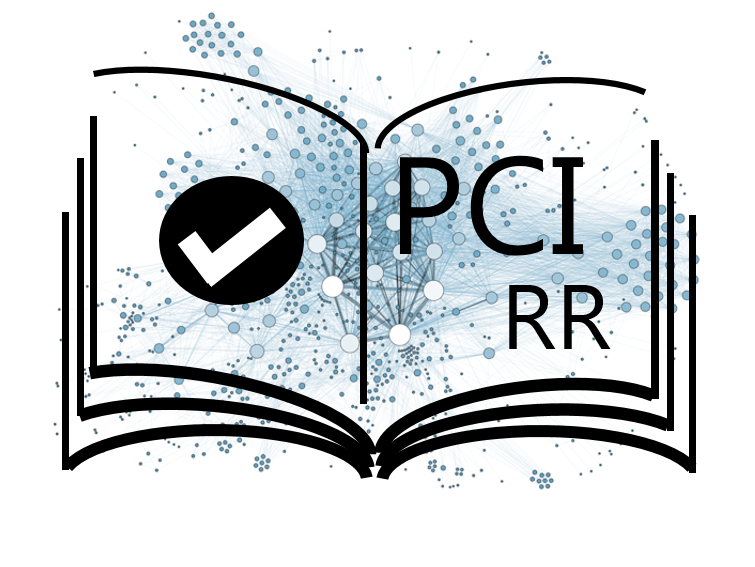
Does familiarity really breed contempt?
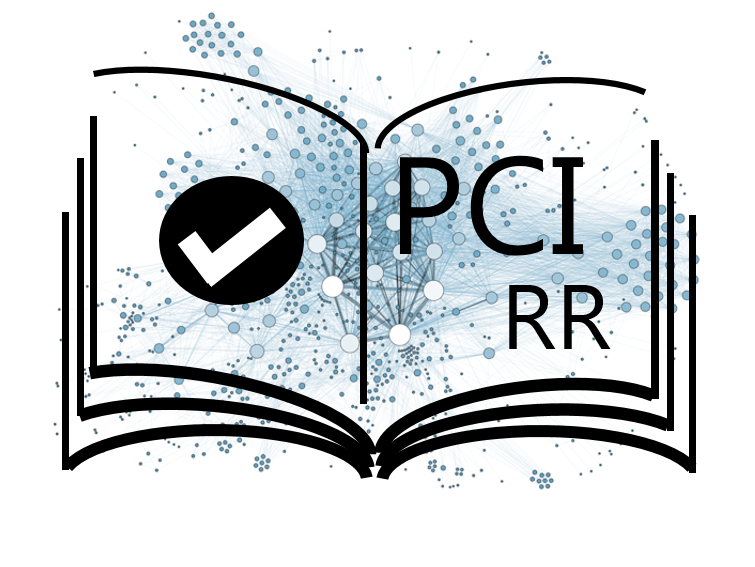
Does learning more about others impact liking them?: Replication and extension Registered Report of Norton et al. (2007)’s Lure of Ambiguity
Abstract
Recommendation: posted 23 May 2024, validated 30 May 2024
Yamada, Y. (2024) Does familiarity really breed contempt?. Peer Community in Registered Reports, . https://rr.peercommunityin.org/articles/rec?id=496
Recommendation
Level of bias control achieved: Level 6. No part of the data or evidence that will be used to answer the research question yet exists and no part will be generated until after IPA.
List of eligible PCI RR-friendly journals:
- Advances in Cognitive Psychology
- Collabra: Psychology
- International Review of Social Psychology
- Meta-Psychology
- Peer Community Journal
- PeerJ
- Royal Society Open Science
- Social Psychological Bulletin
- Studia Psychologica
- Swiss Psychology Open
The recommender in charge of the evaluation of the article and the reviewers declared that they have no conflict of interest (as defined in the code of conduct of PCI) with the authors or with the content of the article.
Reviewed by Zoltan Kekecs
, 22 May 2024
Now I am happy with all of the changes made by the authors.
I would like to thank the authors for their perseverance throughout this review process and for their helpful and detailed responses.
Evaluation round #3
DOI or URL of the report: https://osf.io/ywkqp
Version of the report: 3
Author's Reply, 10 May 2024
Revised manuscript: https://osf.io/eygzp
All revised materials uploaded to: https://osf.io/j6tqr/ , updated manuscript under sub-directory "PCIRR Stage 1\PCI-RR submission following R&R 3"
Decision by Yuki Yamada
, posted 07 May 2024, validated 07 May 2024
We have asked the reviewer to check the manuscript again. As you can see, some minor issues have been raised. I also feel that all these should be resolved before granting an IPA. Please consider them and I would appreciate it if you could revise them again.
Reviewed by Zoltan Kekecs
, 07 May 2024
Review notes by Zoltan Kekecs, PhD
I am grateful for the authors’ detailed response to my suggestions. I have a few further observations and suggestions that may help the authors to improve the study and the manuscript:
- Regarding the order effect: I appreciate the authors’ concern that addressing the order effect in formal statistical inference would create unwanted complexity to the situation, which could threaten the confirmatory nature of this investigation. But instead of doing a confirmatory analysis on the order effect, I simply suggest to do an exploratory sensitivity analysis and/or some other investigations that could hint at the effect of study presentation order. I especially don’t like the authors current proposal that the order effect analysis would only be done if the effect was not confirmed. This practically “stacks the deck” in favor of the authors. If they find the effect, no further investigation is done (which could question the authors’ interpretation), but if the effect is not found, an analysis of the order effect could still salvage the situation and gives an extra chance for finding the effect. I suggest that the authors simply state that an exploratory analysis will be undertaken to investigate the possible influence of order of presentation. This exploratory analysis could include visual analysis of graphs plotted according to presentation order, and displaying descriptive statistics by order of presentation. These graphs and figures could be included in a supplement if these are too big to include in the main article. As the authors say, these analyses are straightforward and do not require too much effort, and this way they also don’t threaten confirmatory power.
- Sample size rationale: I am happy that the authors have revised their power analysis and now provide reproducible R code to support the sample size rationale of their proposal. I would like to point out that the sample size rationale in the current version of the manuscript is still inconsistent. The authors say that “multiplying the largest required sample size among all target studies (208) by 2.5 to 723”. However, 208 x 2.5 = 520.
- It seems that the authors have re-classified H3 as an exploratory analysis. However, this is not properly reflected in the current version of the manuscript. Please, explicitly state in the main text that this research question is not a confirmatory hypothesis, rather, this will be an exploratory analysis. I would also not characterize this as a hypothesis (“H”) anymore, since no inferential statistics should be run on exploratory analyses.
Evaluation round #2
DOI or URL of the report: https://osf.io/m6c7w
Version of the report: 2
Author's Reply, 22 Apr 2024
Revised manuscript: https://osf.io/ywkqp
All revised materials uploaded to: https://osf.io/j6tqr/ , updated manuscript under sub-directory "PCIRR Stage 1\PCI-RR submission following R&R 2"
Decision by Yuki Yamada
, posted 05 Mar 2024, validated 05 Mar 2024
Thank you so much for submitting your revised manuscript.
Two reviewers have checked it again, and as you can see, one reviewer is satisfied with the revision.
Another reviewer is still commenting on the data analysis and power analysis. I hope the authors would address this again.
Reviewed by Zoltan Kekecs
, 26 Feb 2024
Reply to Response #1:
I don’t find most of the arguments by the authors very convincing in why a joint design is needed other than increased sample size. The additional insight in the exploratory research questions is nice, but in a confirmatory RR, these are secondary to being able to adequately address the main effect.
However, I am still satisfied with the action the authors took with one small request. The authors say that “We therefore pre-register that if we fail to find support for our hypotheses that we rerun exploratory analyses for the failed study by focusing on the participants that completed that study first, and examine order as a moderator. “ Maybe the authors misunderstood my comment to mean that I was afraid that the effect would be masked by combining the studies. On the contrary, I am afraid that the effect would only be due to running the studies together. I would like to ask that they re-run the analysis regardless whether the main hypothesis was confirmed or not by focusing on the participants that completed that study first. This way it will be revealed if the effect is only due to combining the studies.
Reply to Response #7:
This reviewer note was about target sample size. The authors say that they intend to analyze all valid cases, and say that they “see no reason to worry about or suspect optional stopping”. Nobody is “worried about optional stopping” before they started collecting data for their own study. Everyone is the hero in their own life’s story. Nevertheless, having clear stopping rules and pre-specified analyzed sample size targets still make sense to prevent conscious or unconscious biases in research. The study is already well powered, with considerable slack. I still suggest that the authors only analyze the data from the first 800 valid responses in their confirmatory analyses. In the exploratory, anything goes.
Relatedly, I would also like to ask the authors to specify the exclusion criteria from the analysis. I could not find now in the manuscript what are the planned exclusion criteria, although 10% exclusion is accounted for in the sample size rationale.
Reply to Response #8:
This note was related to the power to detect all effects, if you have multiple tests and plan for 90% power to detect each effect separately. The authors reply that this is not common practice, and that the community on X was also divided.
Most of the detailed responses you got on X seemed to agree with the point. (Others seemed to misinterpret the question and responded about alpha adjustment, which is not really an issue here).
The important thing is that this is a mathematical necessity. You can calculate this on a napkin, or in R. Simply run a simulation of a study having two effects (with the same effect size and being independent of each other to simplify things), and a sample size powered to be 90% powerful to detect any one of these effects. When you look at how many times you were able to detect both effects, you will find that the probability is 81%. As some posters on X point out, this 81% is a “worst case scenario”, because if the effects do correlate, you will have a correspondence of when you are able to find them, so you power to detect all effect will be closer to the individually calculated powers.
Here is a simple simulation showing the issue: we are simulating 5 effects independent from each other, with a sample size enough to detect each effect 90% of the times. However, in any study, there is only about 59% chance for all of the 5 effects to be significant: https://github.com/kekecsz/power_to_detect_all/blob/main/power_to_detect_all.R
All I am saying is, that in a study where power is set to 90% to detect each effect, the study will have a lower chance to detect all effects in the study.
“Note: We would be happy to revise given clear editorial guidelines and instructions on what to amend. If the reviewer or editor feel that an adjustment in sample target is needed - then we ask that you please provide us with relevant citations and an example or two of other Registered Reports (preferably PCIRR, preferably replications) that has done something similar, and taking into consideration cost/benefit of going beyond the already large planned sample of 800.” – I find this request unnecessary. This mathematical fact does not require citation in my view, since it is easy to demonstrate (see code above)(although the responses on X did contain some useful works if you are interested).
I suggest the authors add a paragraph in the power analysis section, that says something like this: “It is worth noting that even though the power for this study to detect each hypothesized effect is at least 90%, the power of this study to detect all of these effects simultaneously is unknown.” (If you don’t like “unknown”, here you can give the worst-case scenario estimate as I mentioned above, or, if you have reliavble pilot data, calculate the tue power based on the dependency of the effects from there. For all the effects in this study with various effect sizes this might b a complicated calculation, maybe easiest to do with simulation).
Relatedly, the authors say in this sentence: “We conducted a series of a priori power analyses based on these effect sizes and we found that 234 participants would be enough to detect the effect sizes with 90% statistical power at alpha = .05 (see supplementary materials and analysis code for more details).” I don’t understand why the authors say 234. In the PCIRR Study Design Table they say “Based on the reported correlations between knowledge, similarity, and liking (Study 3 in Norton et al., 2007), we conducted a power analysis. It revealed that N = 310 and 400 would achieve statistical power of 80% and 90% respectively to detect the interaction effect.” Shouldn’t the authors have used 400 instead of 234?
I found all other responses by the authors adequate and have no other issues about the registered report.
Reviewed by Philipp Schoenegger, 05 Mar 2024
The authors have responded to all my comments, either directly changing their manuscript properly in response or explaining why they did not follow my recommendations. Why I do not persoanlly agree with all their reasoning in cases where they chose not to follow my recommendations, I can see their point of view, with the remaining disagreements not being scientifically important.
I am thus happy to recommend the Stage 1 for acceptance and am looking forward to seeing the results!
Evaluation round #1
DOI or URL of the report: https://osf.io/4sejv
Version of the report: 1
Author's Reply, 20 Feb 2024
Revised manuscript: https://osf.io/m6c7w
All revised materials uploaded to: https://osf.io/j6tqr/, updated manuscript under sub-directory "PCIRR Stage 1\PCI-RR submission following R&R"
Decision by Yuki Yamada
, posted 06 Sep 2023, validated 07 Sep 2023
First, I apologize that it has taken somewhat longer to collect the peer review reports. The reviewers all submitted their reports very quickly after accepting our request. What took time was the rest of the process, for which I bear the responsibility.
Now, I have just received very helpful peer review comments from two experts. As you can see, both are very positive about this study. And at the same time, they focus on almost the same aspects: power analysis and adjustments for test multiplicity. Please see their specific comments, but I believe their points are in line with current standards of research practice. I encourage you to carefully consider them. The reviewers also made some really constructive comments on the wording of the text, so please take those into consideration as well.
I very much look forward to receiving your revised manuscript!
Reviewed by Zoltan Kekecs
, 16 Aug 2023
Review by Zoltan Kekecs, Phd:
The manuscript describes the protocol for a replication of Norton et al. (2007)’s lure of ambiguity effect. The registered report is thorough and shows not only the protocol but also the results for a simulated scenario. The replication attempt makes a reasonable effort at testing the replicability of the critical results of Norton et al. 2007, and includes extensions to the original research questions that help further evaluate the mechanisms underlying the effect. I really like that materials, data, and analysis code used to produce the manuscript are made openly available by the authors, enabling a thorough evaluation of the work. All in all I think this is going to be a valuable project that has a good chance to replicate the effects if they exist and that can provide deeper insight into the influencing factors and mechanisms at play. Below I list a number of suggestions that may help the authors improve the manuscript and the protocol.
- It seems to me that all participants will do all experiments. However, the subsequent experiments might influence each-other. For example a person who first claimed that they think more traits lead to more liking might respond accordingly in the second study to make their responses more consistent. It seems sensible to me to at least separate Study1 and the rest of the studies to prevent such effects.
- “However, we found the choice of analytic strategies somewhat arbitrary; to directly test the effect of the quasi-experimental condition on liking, it is sensible to conduct a t-test rather than computing the correlation. Thus, while we aimed to replicate the correlation, we also planned to test the relationship with a t-test to see whether the quasi-experimental condition influenced liking.” – There is no point in replicating an inappropriate analysis, especially that this is already a conceptual replication. You should use the best analysis method available to answer the research question.
- I really like the fact that the analysis codes and power analyses codes are available.
- I don’t see why there is not sample size calculation (power analysis) for H2-2 and for H4-3. Instead of effect sizes provided in the original study (not available in this case), you can use smallest effect size of interest, or some other effect size estimation method to gain the required numbers. Simulation can also help. You can do a simulation-based power analysis (of course that would also require setting effect sizes and variances for the simulation).
- In the power analysis for H3 the authors write: “Since the paper does not offer information about standard deviations, we assumed they were 1 and conducted the analysis.” This seems arbitrary. I am not a domain expert so I do not know whether this is a reasonable assumption. This should be supported somehow, for example data from another study, or data from a pilot study. Alternatively, a range of reasonable SDs could be tried in this analysis and the range of estimated samples sizes could be reported in the paper.
- “…the data of the 30 participants will not analyzed other than to assess survey completion duration, feedback regarding possible technical issues and payment, and needed pay adjustments. Unless in the case of serious technical issues that affect data quality and require survey modification, these participants will be included in the overall analyses” These two statements seem to be contradictory. Please reconcile.
- The Participants and design section indicates that 1383 participants were included in the data analysis. This is much higher than the target sample size. Why is this the case? If you exceed the target sample size, it may seem as optional stopping, collecting data until you get the desired results. I suggest that for the confirmatory analyses you only take into account the first X responses, X being the target sample size. If you want you can repeat the analyses on the full sample as a robustness check/sensitivity analysis. Also, the exploratory analyses can be conducted on the full sample.
- You have marked H5 through H9 as exploratory “hypotheses”. Something is either an exploratory analysis or a confirmatory hypothesis test. In the “Extensions” section you use inferential statistics and confirmatory hypothesis testing language for these analyses. Please, decide whether these are exploratory analyses or confirmatory hypothesis tests. If exploratory analyses, do not use p-vlaues, or testing language. Just focus on the descriptive results, effect size estimates, dispersion statistics, and visualization of these. If they are confirmatory tests, do not mark them as exploratory, and provide sample size calculation (power analyses) for these as well.
- The power analysis provides sample size targets to reach at least 90% power for each replication hypothesis tests individually. This seems to assume that you will have at least 90% power to detect the effects in this study. However, this is incorrect, since you are testing multiple hypotheses. For example if you have two hypotheses and have a 90% power to detect each effect, you only have 81% probability to detect both effects, and thus, 19% probability to miss at least one of them. With more hypotheses, this missing effect chance can stack up quickly. You could either power your study to have a 90% probability to detect ALL effects, or be explicit about the probability of missing a number of effects in the Power and sensitivity analysis section.
- „We did not include Studies 3 and 5 as targets of direct replications as these involved experiments using real online dating platforms” – do you mean 4 instead of 5? study 5 ws not mentioned until this point.
Reviewed by Philipp Schoenegger, 06 Sep 2023
The paper under review provides a commendable effort in directly replicating Norton et al. (2007). The authors have done an excellent job in motivating the study and setting up the project. The manuscript is not only well-structured but also remarkably transparent, with a plethora of resources and data made available at the OSF. The research question and hypotheses are clearly articulated, and the methods employed are appropriate. Furthermore, the manuscript is characterized by a high level of detail, making it easy for the reader to follow the research process and understand the nuances of the study. However, I have a number of points that I would like to see the authors address before running the study. Some are minor while others are major, but I believe that this study is very much worth running and would be a great addition to the scientific record. The former are suggestions that may be disregarded with some reasonable explanation, but the latter should be followed or at least be rejected with a detailed argumentation as to the reason for doing so.
1) For the abstract, the use of ‘results’ seems too strong to me, especially given the fact that you use much more associational language throughout the paper. I would suggest you use more uniform language to avoid misunderstandings.
2) Additionally, the term ‘less liking’ should be set-up better in the abstract, a less informed reader may not be able to follow.
3) There is also a small typo in the abstract, it should be ‘Overall, we found’. I generally suggest reworking the abstract for clarity.
4) In the introduction, I would improve the set up early on somewhat better motivate the term ‘stranger’. It seems to me that one may not be able to meet the same stranger regularly (without that person not becoming a stranger anymore). At least this may be the case with the standard definition.
5) Additionally, it is worth keeping an eye on consistent writing of the ‘less is more’ effect. This can be with ‘ or cursive, etc. Just keep it consistent.
6) In the ‘Target for Replication’ section, I would again point out that there is a stark difference in the presentation of the results, particularly in the language used to describe the findings. The manuscript alternates between associational language, such as 'tended to report,' and causal language, represented by terms like 'results.' This inconsistency could lead to confusion regarding the level of causality that the study aims to establish. I suggest adopting a more cautious and consistent approach to causal language. Specifically, it would be prudent to decide on a uniform level of causality that the study aims to establish and maintain this consistently throughout the manuscript. This is particularly important because some of the studies that you are replicating or referring to are associational in nature. Using inconsistent or overly strong causal language could risk misleading interpretations and should therefore be avoided.
7) When you write that “Ullrich et al. (2013) also challenged Norton et al. (2007),” I would change it to a phrasing that suggests that a paper or finding is challenged, not a set of authors.
8) Lastly, in the ‘Conceptual replications of Study 3 and 4’ section, I think your treatment of the conceptual replication of Study 3 could use more detail. I suggest you explain why you do not replicate Study 3 (I assume because of the specific sample, but simply having a different sample is not reason enough, the reason may be cost, temporal differences, effort, etc), and what potential differences this change in sample may bring with it with respect to interpreting any given results.
Below I outline my three bigger concerns:
1) In the methods section, while the inclusion of a power analysis is commendable and aligns with best practices in research methodology, there are several issues that need to be addressed. Firstly, the code provided for the power analysis is not immediately executable without minor modifications (at least for me). For instance, the line of code “esc_chisq(chisq = 112,67, totaln = 294, es.type = "r")” contains a syntax error; the comma should be replaced with a period to read “112.67” instead of “112,67.” Although I was able to replicate your final sample size of 234 participants after making these adjustments, the code should be cleaned up to ensure that it runs seamlessly for other researchers who may wish to replicate or extend your work.
2) Secondly, the choice of approach for determining effect sizes in the power analysis warrants discussion and perhaps revision. The manuscript seems to rely on expected effect sizes derived from a single paper, which is a methodological choice that could be problematic. Given that the very essence of replication studies like this one is to question the generalizability of such expected effect sizes, it would be more prudent to adopt a 'smallest effect size of interest' approach instead of using the observed effect size as the expected effect size. This would involve identifying the smallest effect that would still be of scientific interest and using that as the basis for the power analysis, rather than relying on potentially inflated or context-specific effect sizes from previous work. The 'smallest effect size of interest' could be anchored initially to the expected effect size but should be revised downwards to reflect a more conservative and scientifically rigorous estimate. This approach would align better with the overarching goals of replication studies, which aim to rigorously test the robustness and generalizability of previous findings. If the authors disagree with this suggestion, a detailed justification for the current approach would be beneficial, including why it is considered superior or more appropriate for this specific study. I am also aware that this is likely to reduce the effect size and thus increase the sample size needed, but for an important replication like this, these additional costs seem justified and indeed crucial.
3) Lastly, I noticed that the 'Results' section (or any other place) does not include adjustments for multiple hypothesis testing. Given that this is a replication study with multiple hypotheses, it would be beneficial to consider some form of adjustment to maintain the integrity of the results. I checked the analysis code and, as far as I can tell, found no evidence of any such adjustments that may not have been mentioned in the paper, with any instances of 'p_adjust' set to 'none.' I would strongly suggest that the authors consider incorporating a method to correct for the multiple hypotheses being tested in this study.
Section Results: Lastly, I am surprised not to see any adjustments for the multiple hypotheses that are tested in this replication. I went to the analysis code to ensure it wasn’t just left out of the manuscript but I could find no adjustment either, with the few instances of p_adjust set to none. I would strongly suggest that the authors include some type of adjustment that corrects for the large number of hypotheses tested in this replication.