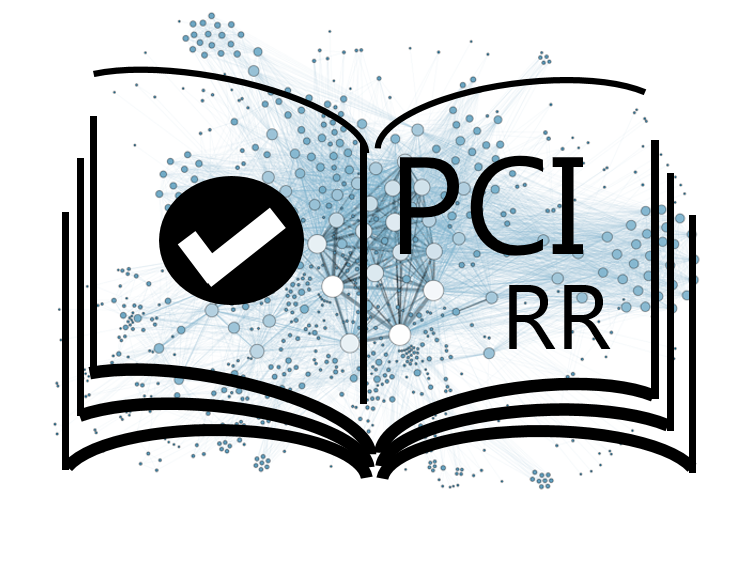
Examining the causal pathways from impulsivity to disordered gambling in online sports betting
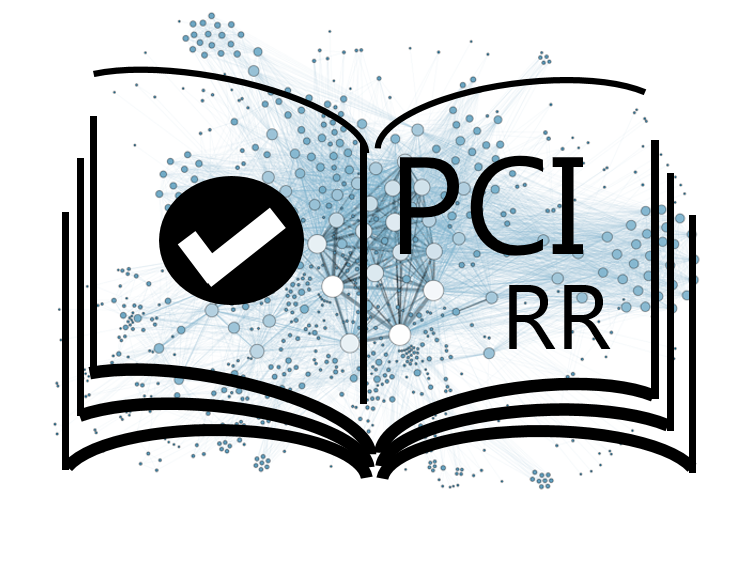
Impulsivity and online sports betting behaviour: Untangling the causal relationship (ISPO study)
Abstract
Recommendation: posted 27 March 2024, validated 27 March 2024
Chen, Z. (2024) Examining the causal pathways from impulsivity to disordered gambling in online sports betting. Peer Community in Registered Reports, . https://rr.peercommunityin.org/articles/rec?id=574
Recommendation
Impulsivity has long been recognized as a risk factor for disordered gambling, yet how impulsivity may lead to gambling-related harms is not entirely clear. In the current study, Jacob and colleagues (2024) aim to examine this question using a longitudinal cross-lagged panel design. Regular online sports bettors will be invited to fill out measures of impulsivity (including impulsive choice, impulsive action, and impulsivity personality) and gambling disorder symptoms three times at three-month intervals. Player tracking data on these players' online betting behavior in these three-month intervals will then be retrieved from the online gambling provider. Combining these data provides a unique opportunity to test the hypotheses that impulsivity may lead to riskier online gambling behavior, which in turn may lead to more gambling-related problems later on. The results of the current study will offer novel insights into the causal pathways from impulsivity to disordered gambling in online sports betting, and may also inform the development of early prevention strategies to reduce gambling-related harms.
The current Stage 1 manuscript was evaluated by two reviewers and the recommender. Based on detailed responses to the reviewers' comments, the recommender judged that the manuscript met the Stage 1 criteria and therefore awarded in-principle acceptance (IPA).
Level of bias control achieved: Level 3. At least some data/evidence that will be used to the answer the research question has been previously accessed by the authors (e.g. downloaded or otherwise received), but the authors certify that they have not yet observed ANY part of the data/evidence.
List of eligible PCI RR-friendly journals:
- Addiction Research & Theory
- Peer Community Journal
- PeerJ
- Royal Society Open Science
- Swiss Psychology Open
- WiderScreen
1. Jacob, A., Joshanloo, M., Czernecka, R., & Kräplin, A. (2024). Impulsivity and online sports betting behavior: Untangling the causal relationship. In principle acceptance of Version 4 by Peer Community in Registered Reports. https://osf.io/7wsfh
The recommender in charge of the evaluation of the article and the reviewers declared that they have no conflict of interest (as defined in the code of conduct of PCI) with the authors or with the content of the article.
Evaluation round #3
DOI or URL of the report: http://dx.doi.org/10.23668/psycharchives.14222
Version of the report: 3
Author's Reply, 25 Mar 2024
Dear Dr. Zhang Chen,
We are very pleased that you noticed this error. We have carefully double-checked all the contents of Table 1 and adjusted it according to the previous changes in the manuscript in revision round 2.
Furthermore, we now also present the status of the current recruitment (2 out of 3 waves completed) and have added a CREDIT statement to the manuscript. We have again submitted a revised manuscript with tracked changes.
We thank you for all your constructive feedback!
Kind regards
Anja
Decision by Zhang Chen
, posted 20 Mar 2024, validated 20 Mar 2024
Dear Dr. Kräplin,
Thank you again for the opportunity to read your revised manuscript "Impulsivity and online sports betting behavior: Untangling the causal relationship". All my previous comments have been addressed very satisfactorily.
During my final read of the whole manuscript, however, I noticed that some content in Table 1 has not been updated to match the revised manuscript. For instance, for the data analysis plan for Hypothesis 2, the method for determining the number of factors for risky gambling behavior seems to be based on an old text, and there is no mentioning of setting a maximum number of factors in the analysis. It would be good to resolve this inconsistency (and perhaps have a look at other cells in Table 1 as well), since once this Stage 1 manuscript is accepted, there will be strict limits on changing the accepted content.
I would be happy to recommend this manuscript once this minor revision has been made.
Kind regards,
Zhang Chen
Evaluation round #2
DOI or URL of the report: http://dx.doi.org/10.23668/psycharchives.14187
Version of the report: 2
Author's Reply, 08 Mar 2024
Decision by Zhang Chen
, posted 01 Mar 2024, validated 01 Mar 2024
Dear Dr. Kräplin,
Thank you for submitting your revised Stage 1 Registered Report, entitled "Impulsivity and online sports betting behavior: Untangling the causal relationship" to PCI RR. All previous comments have been addressed very satisfactorily. Both the procedure and data analysis plans are much clearer now. However, there are some final issues that still need to be further clarified:
The methods for determining the number of factors in risky betting behavior are now made concrete (starting from line 411 in the revised manuscript). However, in the Hypothesis testing section, starting from line 554: "If the eigenvalue of the first factor is significantly larger than those of the remaining factors, we will extract a single factor, resulting in a single risky gambling behavior score per participant. If inspection of the eigenvalues indicates several factors, we will run five separate models (5 predictors) for each of the factors." This method of determining the number of factors seems inconsistent from the section above (line 411). Please clarify this point.
I am afraid the statistical inference process for Hypothesis 2 is not entirely clear to me. If the factor analysis reveals multiple factors for risky betting behavior, say 3, I assume 15 models will be run in total for Hypothesis 2. Will a sub-hypothesis be considered to be supported if it correlates with at least one of the 3 factors on risky betting behavior? If that is the case, the test for a sub-hypothesis seems to become less stringent as the number of factors for risky betting behavior increases. I am wondering if you have considered alternatives, for instance, by requiring a predictor to be associated with at least N-1 factors (in case N > 1) of risky betting behavior, in order to say a certain sub-hypothesis is supported. I guess this will also be consistent with how you deal with the three facets of impulsive personality at the moment, by requiring at least two of them to correlate with outcomes of interest.
For Hypothesis 3, I wonder whether all models will be run, or only a subset of them will be examined depending on the results of Hypothesis 1 and 2. For instance, will the potential mediation effect of a certain factor of risky betting behavior still be examined, if the analysis in Hypothesis 2 revealed that it was not predicted by impulsivity?
Minor point: line 53: "an increase of 15,6%". You may want to use "15.6" to be consistent with the rest of the manuscript.
Kind regards,
Zhang Chen
Evaluation round #1
DOI or URL of the report: https://doi.org/10.23668/psycharchives.13476
Version of the report: 1
Author's Reply, 23 Feb 2024
Decision by Zhang Chen
, posted 01 Feb 2024, validated 01 Feb 2024
Dear Dr. Kräplin,
Thank you for submitting your Stage 1 Registered Report, entitled "Impulsivity and online sports betting behavior: Untangling the causal relationship" to PCI RR. I have now received comments from two expert reviewers, and have also read the manuscript myself. Combining player tracking data and survey data provides a unique opportunity to understand the etiology of gambling disorder, which is also a key strength of the proposed research. However, my major concern with the current manuscript is that certain methodological details do not seem to be fully specified, which can leave room for potential undisclosed flexibility in the procedures (see the review criteria 1C and 1D on the website of PCI RR). The reviewers and I have noted several aspects where more clarity is needed to reduce such potential flexibility. I hope these comments will be useful to you, and I look forward to seeing a revised version of the manuscript.
Please provide more information for the power analysis, such as: the exact statistical model used in the simulation, the assumed effect sizes (at the moment, the text only says "small associations" and "a small to medium association", without giving any specific values), the criterion for statistical inference in the simulation (an alpha level of .05?), and the observed power for each of the three hypotheses (I assume they will differ for the three hypotheses?). Such information will help readers better appreciate what effect sizes the target sample size will have a reasonable chance of detecting.
Both reviewers have concerns about whether the target sample size of 370 can be reached given the sample size at the first wave. This is of course an inherent difficulty with such a longitudinal design - one can never know in advance what dropout rate will eventually be observed. That the initial sample size at wave 1 slightly exceeded the planned sample size, and the dropout rate observed in a past study with a similar design to some extent support the feasibility of achieving the target sample size at wave 3. It would nevertheless be good to know if there are any contingency plans in place to address this potential issue.
Related to the previous point, since data collection for wave 1 is already finished, achieving a low dropout rate for waves 2 & 3 seems to be key here for reaching the target sample size. More methodological detail on the recruitment procedure would be helpful in this regard. For instance, how much time did/would participants have to participate in the task after getting an invitation? Did/would participants get reminders if they did not respond to the initial invitation, to potentially reduce the dropout rate for waves 2 and 3? In general, more details could be provided for the recruitment procedures for all three waves, to give a better picture of how the recruitment took place/will take place.
Please specify how the balanced integration score will be calculated. I think it will be more convenient to the readers, and also reduces potential flexibility in data analysis, if they do not need to look up another source to understand this method.
Please also specify how the scores for impulsive personality traits will be computed. For instance, will the sum score, average score, factor score (or an alternative score) be used for each of the three factors?
More clarity is needed for how the variables operationalizing riskier gambling behavior will be constructed. For instance, for "a higher variance (mean standard deviation) in the number of bets and stakes over 3 months", will the number of bets/stakes first computed per day, or per week, or per month, before the standard deviation is computed? For "betting at higher odds (mean weighted odds) over 3 months", how will the odds be weighted exactly? For "no or low gambling limits set by the player over 3 months", what constitutes "low limits" in this case?
To promote transparency, please consider sharing the survey used in this project. Please also describe the procedure in the survey for all three waves (e.g., in which order do participants receive the different tasks and questionnaires etc.). Will the same plausible time limit (i.e., 10 minutes) also be used for waves 2 and 3?
Line 354: "we expect some individuals with too few data points to compute a reliable aggregated variance". What criterion will be used to say that a certain individual has "too few" data points?
As one reviewer pointed out, please specify how the number of factors will be determined for the variable risky gambling behavior. At the moment, the description (lines 372 - 377) seems to largely rely on subjective judgment, which leaves much room for flexibility in data analysis.
For H1, five separate models will be run. For H2 and H3, potentially more models will be run, depending on the number of factors obtained for the variable risky gambling behavior (which needs to be further specified, see above). This raises the question of how the results of these separate models will be used to infer whether a certain hypothesis is supported or not. For instance, will H1 only be considered to be supported when all 5 models reveal an effect of impulsivity on gambling disorder severity, or will the different facets of impulsivity be considered separately? If it's the latter case, the hypotheses will also need to be adjusted accordingly to reflect that the statistical inference will be made for each facet (instead of impulsivity as one construct). The same applies to H2 and H3.
Multiple indices will be used to assess model fit. Please specify how these indices will be used in the statistical inference. For instance, will the results from a model only be interpreted if all (or if any) of the indices show that there is adequate fit? Differences in model fit will also be computed, but it is not entirely clear to me which models will be compared, and what conclusions will be drawn from such model comparisons.
Line 280: "lack of consciousness" should be "lack of conscientiousness"?
Kind regards,
Zhang Chen
Reviewed by anonymous reviewer 2, 23 Jan 2024
See attachment
Download the reviewReviewed by anonymous reviewer 1, 24 Jan 2024
To the authors:
Below are my comments as of Stage 1. I hope you find them constructive.
----------------------------------------------------------------------
Abstract, math typesetting: Add spaces between equal signs and "n" and other variable letters. n = 370.
Introduction, growth of sports betting: I noticed that you're using commas for things like 0-point-3 billion. It should be written as "$0.3 billion in revenue."
Introduction, "Researchers and policymakers are concerned...": If I remember correctly, it's the increase in online sports betting _availability_ that people are concerned about. Granted, it's because the increase in availability and increase in legal venues that creates the increase in participation, which might lead to problems at the individual and population levels.
Introduction, hypotheses: Here and throughout the report, switch to active voice and take ownership of the methodology. "We proposed three directional hypotheses to test our main assumption."
Participants, sample size, response rates and dropout rates: You're looking for an ending sample size of n3 = 370, and you expect to keep 40% of those you start with in Wave 1. Assuming that whether one person drops out is independent of whether anyone else drops out, if n1 is the number of people you start with in Wave 1, then n3 has a binomial distribution with n1 trials with probability of success 0.4. Semi-ideal would be a 95% chance that n3 is greater than or equal your target of 370. (n1)(0.4) - 1.645*sqrt(n1*0.4*0.6) = 370. Solve for n1... I'm getting 989. Your calculations have a 0 where I have -1.645, so according to this model, you've got a 50/50 chance of getting the final sample size you want. Your sample size of n1 = 925 might be a bit short, you might get lucky, or you might have a plan to decrease that 60% dropout rate.
Recruitment, actual n1: You have n1 = 954. With that 40% finishing rate, you've got about a three-quarters chance of ending with 370 or more at Wave 3.
Measures, factor analysis of gambling measures: What threshold will you use to determine how many factors you will use in your models? Just the eigenvalues? What will you do if the first factor explains, for example, only 10% of the variation between gamblers?
Statistical analysis, factor analysis: Consider including a rundown of the factors and which gambling measures are most salient. You might be able to add descriptive labels to the factors. You might be able to separate the gamblers into different groups based on whether they're extremely high in one factor or extremely low in another. For example, one factor might heavily lean on amount wagered on in-game bets, and there might be people who have an extremely high value for that factor (in-game betting specialists?) and people who have a value closer to zero (pure pre-game bettors?).